Artificial Intelligence
Stanford Unveils AI Tool for Finding Brain Aneurysms
Deep learning helps clinicians identify brain aneurysms with better accuracy.
Posted June 11, 2019
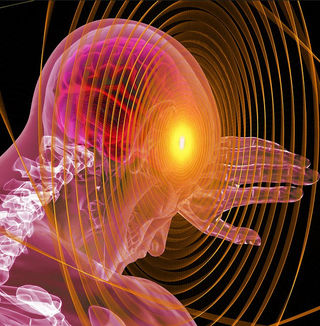
Brain aneurysms grow silently and can be deadly. An aneurysm is when the wall of an artery is weakening, causing it to bulge or widen abnormally. Globally, nearly half a million people die each year due to ruptured brain aneurysms, and an estimated six million people in the U.S. have an unruptured brain aneurysm according to statistics from the Brain Aneurysm Foundation.
When brain aneurysms rupture, 40 percent of cases end in death, and for those that do survive, 66 percent are left with lasting neurological damage. Diagnosing aneurysms early is challenging because it can develop slowly, and without causing any symptoms. Recently, researchers at Stanford University announced a new non-invasive diagnostic tool that uses artificial intelligence (AI) deep learning to help clinicians identify unruptured brain aneurysms from medical images with greater accuracy.
The Stanford research study, with co-senior authors Kristen Yeom, associate professor of radiology, and Andrew Ng, adjunct professor of computer science, and co-lead authors Allison Park, Chris Chute, Pranav Rajpurkar, and their collaborators, was published on June 7, 2019 in JAMA Network Open, an international peer-reviewed open access general medical journal.
The researchers created HeadXNet, a three-dimensional (3D) deep learning convoluted neural network (CNN), which is a type of neural network used to identify and analyze images.
Computed tomographic angiography (CTA) is a medical test where the patient is injected with a dye intravenously in order for blood vessels and tissues to illuminate on an x-ray that uses a computer for cross-sectional imaging called a CT scan (computerized tomography scan).
HeadXNet uses CTA image data to produce predictions for each voxel. In computer graphic modeling and simulation, voxels are graphic units in 3D—one dimension more than two-dimensional pixels.
This study used retrospective data from the CTA exams of the head and neck area from Stanford University Medical Center patients during the period 2003-2017. Out of 9,455 CTA scans, 818 eligible CTA scans (328 aneurysms, 490 normal) were selected with exclusion criteria validated by a board-certified neuroradiologist. From the 818 CTA scans, 611 was used for the training set, 92 for the development set, and 115 for the test set.
To train HeadXNet, the researchers underwent the time-consuming manual task of labeling each voxel, regardless if it was part of an aneurysm, in 611 head scans performed by computed tomographic angiography.
The model was trained on three NVIDIA TITAN Xp GPU (graphical processing units). The massively parallel processing power of GPU technology, along with breakthroughs in deep learning algorithms, has revived artificial intelligence from relative dormancy and contributed to the current AI renaissance.
The deep learning model was optimized using stochastic gradient descent (SGD)—an iterative way to find the optimal parameter configuration for a machine learning algorithm. Stochastic gradient descent is faster to calculate than a true gradient descent—it approximates the gradient for a randomly selected (stochastic) batch rather than the complete data set.
HeadXNet was put to the test with eight clinicians consisting of six board-certified practicing radiologists, along with a practicing neurosurgeon and a Stanford University Medical Center radiology resident.
The team of eight clinicians evaluated the test set of 155 CTA scans that contained 59 scans with aneurysms twice—assisted and unassisted with HeadXNet.
During the assisted test, clinicians were shown the model’s predictions as semi-opaque red overlays on top of the CTA scans. On voxels where the model predicted values are greater than 0.5 for an aneurysm, the risk is indicated by an overlay. The clinicians then decided whether or not to use the model’s prediction in their decisions.
According to the researchers, HeadXNet improved the clinicians’ performance in both sensitivity and accuracy. “With augmentation, there was a statistically significant increase in the mean sensitivity (0.059; 95% CI, 0.028-0.091; adjusted P = .01) and mean accuracy (0.038; 95% CI, 0.014-0.062; adjusted P = .02) of the clinicians as a group,” the researchers reported. "There was no statistically significant change in mean specificity (0.016; 95% CI, −0.010 to 0.041; adjusted P = .16)."
“Our results demonstrate that deep learning segmentation models may produce dependable and interpretable predictions that augment clinicians and improve their diagnostic performance,” wrote the researchers. “The model implemented and tested in this study significantly increased sensitivity, accuracy, and interrater reliability of clinicians with varied experience and specialties in detecting cerebral aneurysms using CTA.”
Artificial intelligence is rapidly emerging as an important tool in life sciences and health care, especially in applied deep learning for medical imaging and neuroscience, where manually searching for patterns in vast quantities of highly complex data is extremely challenging and time-consuming. This study shows the feasibility of how AI-based technology might in the future assist health care professionals as a tool to improve the odds of better patient outcomes—for the benefit of humanity.
Copyright © 2019 Cami Rosso All rights reserved.
References
Park, Allison, Chute, Chris, Rajpurkar, Pranav, Lou, Joe, Ball, Robyn L., Shpanskaya, Katie, Jabarkheel, Rashad, Kim, Lily H., McKenna, Emily, Tseng, Joe, Ni, Jason, Wishah, Fidaa, Wittber, Fred, Hong, David S., Wilson, Thomas J., Halabi, Safwan, Basu, Sanjay, Patel, Bhavik N., Lungren, Matthew P., Ng, Andrew Y., Yeom, Kristen W..”Deep Learning–Assisted Diagnosis of Cerebral Aneurysms Using the HeadXNet Model.” JAMA Network Open. June 7, 2019.
Bottou L. (2012) Stochastic Gradient Descent Tricks. In: Montavon G., Orr G.B., Müller KR. (eds) Neural Networks: Tricks of the Trade. Lecture Notes in Computer Science, vol 7700. Springer, Berlin, Heidelberg