Artificial Intelligence
Novel AI Model Predicts Breast Cancer as well as Doctors
IBM Research’s algorithm uses data from both mammograms and health records.
Posted June 18, 2019
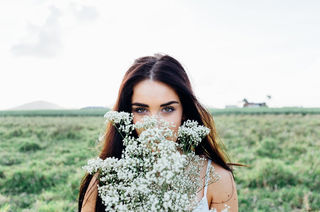
Published today in the peer-reviewed journal Radiology, an IBM Research team created a new artificial intelligence (AI) model that can predict breast cancer malignancy and identify normal digital mammography exams as accurately as radiologists.
Mammography, a low-dose X-ray procedure to image breasts, is considered the best breast cancer screening test available according to the American Cancer Society. However, mammograms are not always accurate. According to a U.S. 10-year study published in the New England Journal of Medicine, 23.8 percent of study participants had at least one false positive mammogram where breast cancer was not actually present. Furthermore, the American Cancer Society estimates that one in five screening mammograms are false-negatives that fail to detect existing breast cancer.
In efforts to improve the accuracy of mammograms, researchers at one of the largest laboratories of IBM Research Division outside of the United States located in Haifa, Israel, created a new AI model to assist radiologists. Specifically, the researchers’ objectives were to predict malignancy and identify normal digital mammography exams. In digital mammography exams (full-field digital mammography or FFDM), solid-state detectors convert X-rays into electrical signals which are used to produce images.
“The uniqueness of the work lies in providing assessment of cancer and normal cases based on a connection between a rich set of clinical features characterizing the woman's health history with the mammography,” said IBM researcher Michal Chorev. “Most, if not all studies thus far, focused only on images or only on clinical data.”
The retrospective study used anonymized data of 52,936 images from 13,234 women who had at least one mammogram during 2013-2017 in one of the five Assuta Medical Centers imaging facilities, and had at least a year of health records at Maccabi Health Services prior to mammography. Three non-overlapping data sets were created. The majority of the data, 73 percent, was used to create a training data set consisting of 9611 women with 38,444 images. The remaining data was used to create a testing data set of 2548 women with 10,192 images, and a validation data set of 1055 women with 4220 images.
The researchers used the XGBoost algorithm, an open-source Python implementation for gradient boosting, to select a subset of clinical features that contribute the most to predicting a biopsy positive for cancer for use in a deep neural network (DNN). An ensemble of deep neural network (DNN) algorithms were trained separately for each prediction objective per digital mammography image (both with and without the subset of clinical features). Imaging features from the bilateral craniocaudal and mediolateral oblique views of the breast along with the clinical feature set were combined to form a single rendering of the patient’s breast. XGBoost was used to estimate the final probability for either a biopsy positive for cancer or normal or abnormal differentiation.
The AI model was able to accurately predict the development of breast cancer in 87 percent of the cases, and correctly interpret 77 percent of non-cancerous cases. Furthermore, the AI model was put to the test on 71 separate false-negative cases where the patient was later diagnosed with breast cancer within the year. The AI model was able to identify breast cancer in 48 percent of the 71 patients. The researchers wrote that the AI model’s “ability to lower false-negative results by half is of immediate clinical relevance.”
“We know that cancer detection and moreover differential diagnosis of cancer—detecting not only that there is cancer but what type of cancer it is and what is the likelihood of a cancer to become metastasis and aggressive—is a challenging open question,” said Chorev. “We would like to extend our algorithms to combine not only clinical history and medical images, but also digital pathology images and potentially genomic data. And we hope to test the promise of AI techniques to not only detect cancer early but to shed some light on the specific disease prognosis. A technology like that, if accurate, can assist in saving more lives while reducing the known phenomena of over-treatment.”
The researchers view their AI model particularly helpful in scenarios when double reading at mammography screening is unavailable. By creating a new type of AI model trained on data with linked health records and mammograms, the researchers have created a cutting-edge breast cancer screening tool to assist radiologists in the pursuit of better patient outcomes.
Copyright © 2019 Cami Rosso All rights reserved.
References
Akselrod-Ballin, Ayelet, Chorev, Michal, Shoshan, Yoel, Spiro, Adam, Hazan, Alon, Melamed, Roie, Barkan, Ella, Herzel, Esma, Naor, Shaked, Karavani, Ehud, Koren, Gideon, Goldschmidt, Yaara, Shalev, Varda, Rosen-Zvi, Michal, Guindy, Michal. “Predicting Breast Cancer by Applying Deep Learning to Linked Health Records and Mammograms.” Radiology. June 18, 2019.
Chorev, Michal. “AI Models Predict Breast Cancer with Radiologist-Level Accuracy.” IBM. June 18, 2019.
Elmore, JG, Barton, MB, Moceri, VM, Polk, S, Arena, PJ, Fletcher, SW. “Ten-year risk of false positive screening mammograms and clinical breast examinations.” New England Journal of Medicine. April 16, 1998.
American Cancer Society. “Limitations of Mammograms.” Retrieved 6-18-2019 from https://www.cancer.org/cancer/breast-cancer/screening-tests-and-early-d…
Stanford Health Care. “Full-Field Digital Mammography (FFDM).” Retrieved 6-18-2019 from https://stanfordhealthcare.org/medical-tests/m/mammogram/digital-mammog…