Artificial Intelligence
Confirmation Bias in the Era of Large AI
How the input is framed can affect the output produced.
Posted May 1, 2023 Reviewed by Michelle Quirk
Key points
- How users interface with passive AIs is different from the way they interface with large language models (LLMS) like ChatGPT and Bard.
- LLMs rely on direct and intentional user input to predict a response that fits the specifications of that input.
- The outputs generated are sensitive to the framing of the input, leading to the potential for confirmation bias to steer the output.
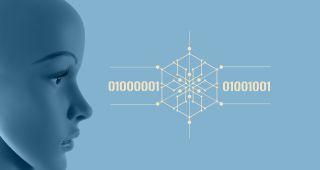
Although ChatGPT, Bard, and other large language models (LLMs) have made headlines recently, artificial intelligence (AI) has been influencing human decision-making for some time. From newsfeeds in Facebook or Twitter to recommendations from Netflix and Amazon, AI takes user input and continuously seeks to use that input to influence users to act (to purchase products, read and interact with posts, and watch movies).
But the AIs that tailor recommendations at Amazon, Netflix, and Twitter largely rely on passive interaction with the user. The user is not interfacing directly with the AI, but the AI is using data from the user’s interaction with the platform to predict ways to influence that user. It essentially operates under the mantra of past behavior is the best predictor of future behavior.1 And in the case of newsfeeds, this can lead to a self-reinforcing echo chamber where users are fed information that aligns with their worldview. This can easily play on user’s confirmation bias, sometimes leading to more extreme or hardened beliefs.
How users interface with more passive AIs, though, is different from the way they interface with LLMs. More passive AIs rely on users’ past behavior to predict how to influence those users (so the user is not interfacing directly with the AI at all), but those AI recommendations are constrained by the availability of products, posts, or movies on the platform.
LLMs, though, rely on direct and intentional user input to predict a response that fits the specifications of that input. The only constraints surrounding the output of the LLM are (1) user-imposed constraints based on the input and (2) the breadth and scope of the data on which the LLM was trained. That means the outputs LLMs can produce are markedly more expansive than the outputs produced by more passive AIs.
But that leads to a few questions:
- If the user can decide how to frame and constrain the input, might those choices influence the quality of the response that is provided?
- Might the outputs generated by LLMs be susceptible to the motivated reasoning of the user?
I posed both questions to Bard and ChatGPT to see what sort of answer each would provide. Though the nuances in the responses varied, both Bard and ChatGPT concluded the answer was yes.2
As I detailed in a previous post, the framing of the input can predispose the AI to generate a response consistent with that framing. The example Bard provided further illustrates how the way a question is worded may alter the output produced:
For example, if a user is motivated to find evidence that climate change is not real, they could ask the LLM "What are the arguments against climate change?" The LLM is likely to give a list of arguments that are consistent with the idea that climate change is not real. However, if the user asked the LLM "What is the scientific consensus on climate change?", the LLM is more likely to give a response that is consistent with the scientific consensus, which is that climate change is real and caused by human activity.
In preparing for this post, I decided to ask ChatGPT and Bard to independently generate arguments in support of two claims:
- Biases aid decision-making.
- Biases harm decision-making.
The results were quite interesting. For the claim that biases aid decision-making, both AIs were able to generate some benefits to biases, such as making decisions more quickly and efficiently and making decisions that align with the decision maker’s goals or values.3 But both ChatGPT and Bard made sure to include that biases are often viewed as negative aspects of decision-making. Bard even went so far as to spend 40.3 percent of its response (205 words out of 509 total words in the response) explaining why biases are detrimental to decision-making.
When I posed the alternate claim, in neither case was anything positive or beneficial mentioned about biases.4 So, even though I attempted to produce an argument that was fully consistent with a particular framing, in both cases, it appears that the AIs are biased (and strongly so) against the benefits of biases for decision-making.5 Still, the results did reveal that the framing of the question affected what was produced. Someone wanting to write an argument praising the benefits of biases would at least be introduced to the idea that biases can also present some problems. However, the flip side was not the case. Someone wanting to argue that biases are a detriment to decision-making would easily walk away with reinforced views about that conclusion with little nuance given to the benefits biases provide.
And for arguments in which the training data don’t lead to the AI producing biased results (such as the GMO example from my earlier post), my experience thus far suggests that user framing plays an influential role in the response that is produced. As such, users should be cognizant of the implications of their input (e.g., underlying assumptions, implied meaning, leading statements), as these may unintentionally influence the output that is produced. But, if their intent is merely to use ChatGPT, Bard, or some other LLM to seek confirmation for an existing view, there’s an increased likelihood that LLM will oblige—as long as that input is framed accordingly.
References
1. Though I regularly question the utility of Amazon’s predictive algorithms, which seem to have a propensity to recommend products I’ve already purchased. (How many leaf blowers does one person need?)
2. Which, of course, I already knew, but I was curious to see if the AIs concurred with that conclusion.
3. ChatGPT also claimed biases can improve creative, outside-the-box thinking, but, to the best of my knowledge, that seems counter to pretty much every claim I’ve seen about biases.
4. ChatGPT’s response began with “While biases may seem like a natural aspect of decision making,” with the sentence ending with “there are several reasons why biases can actually harm decision making.” I did not categorize such a statement as an endorsement of the benefits of biases.
5. This is unsurprising, given the way biases are discussed and communicated in scholarly and more popular press writings, issues I discussed here, here, and here.