Bipolar Disorder
Video Recordings May Help Diagnose Mental Health Conditions
Using audiovisual data to help clinicians and patients.
Posted January 28, 2022 Reviewed by Davia Sills
Key points
- Progress has been made in understanding how best to integrate technology into the field of psychiatry.
- Algorithms might help predict who has schizophrenia or bipolar disorder with high accuracy.
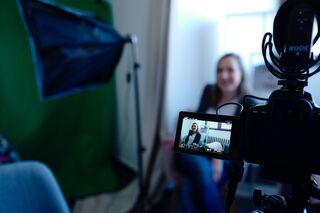
Over the past few years, significant progress has been made in understanding how best to integrate technology into the field of psychiatry. Researchers are developing new tools to diagnose more accurately and efficiently and treat conditions using digital data from social media, online search activity, wearables, and more emerging technology like bioelectronic medicine.
Most of this research aims to provide more objective information to clinicians and patients to improve care delivery and outcomes. Unlike a doctor who can examine a lab value or x-ray to determine treatment recommendations, mental health care relies much more heavily on self-reported data, which is extremely valuable but can also be inaccurate.
A study on using audiovisual data for diagnostic purposes
One exciting advancement that colleagues and I have been exploring is how to use audiovisual data (facial movement and voice) to aid in the diagnostic process. A recent study published in JMIR Mental Health shows promising results in using these features to develop machine learning algorithms that may help predict who has schizophrenia spectrum disorders (SSD) and who has bipolar disorder (BP) with high degrees of accuracy.
Eighty-nine participants between the ages of 15 and 35 enrolled in the study, and 146 interviews were filmed and recorded, capturing facial expressions and voice (tone, pitch, etc.). Participants were asked to tell us about their favorite meal, discuss a television show or movie they recently watched, anything that would get the participants to speak freely for as much time as they liked. The acoustic voice and facial movement data were extracted and used to develop machine learning algorithms to identify distinguishing features and commonalities among the study participants.
Interestingly, the strongest signals separating men with SSD from men with BD were derived from facial features, while the strongest signals for women were derived from acoustic features. For both men and women, participants with SSD were more likely to activate the facial muscle responsible for pulling the corners of their lips than participants with bipolar disorder, which is one of the muscles necessary to form a smile.
This research is exciting because the findings suggest that extracting and analyzing audiovisual data might offer valuable clinical information, supporting a more reliable diagnostic approach, paving the way for better care.
While there is still much more research to be done, the potential for integrating audiovisual data could one day change the way mental health clinicians diagnose and care for patients, enabling quicker and more accurate personalized medicine.