Artificial Intelligence
New AI Tool May Speed Up Drug Discovery Using Images
Japanese researchers use AI to gain insights from cryo-electron microscopy.
Posted February 7, 2021 Reviewed by Abigail Fagan
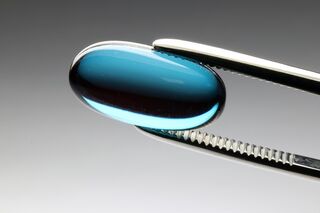
Artificial intelligence (AI) machine learning is transforming pharmaceutical drug discovery. Advances in deep learning, a subset of machine learning, is enabling researchers to identify patterns in fields where there are large amounts of complex data, such as imaging.
In a new study published on Thursday in Nature Machine Intelligence, researchers in Japan created an AI deep neural network to extract information on protein dynamics from images captured using cryo-electron microscopy (cryo-EM).
“Technical breakthroughs in cryogenic electron microscopy (cryo-EM)-based single particle analysis have enabled the structures of numerous proteins to be solved at atomic or near-atomic resolutions, including extremely large macromolecules whose structures could not be solved by conventional techniques,” wrote the researchers. “Determining the dynamics properties of these macromolecules, based on their solved structures, can further improve our understanding of their functional mechanisms.”
Many drugs work by binding to a target protein in order to alter its function. Most drug discovery is done using a target-based (versus phenotype-based) approach where scientists hypothesize which nucleic acids or proteins to target to treat specific diseases and conditions.
Chemical compounds are enhanced for a variety of reasons, including to decrease toxicity, improve solubility, and increase affinity to the target protein. Having a map of the scaffold of the protein binding site accelerates the understanding of how to enhance compounds for desired characteristics.
With knowledge of the three-dimensional protein structure and crystallization condition of a chemical compound, scientists can co-crystallize it with the target protein in order to get the architecture of the protein-ligand.
In biochemistry, a ligand is a molecule, ion, or single atom that binds to a protein in a manner that can be reversed. Ligands can occur in nature or be manufactured synthetically in a lab. When ligands bind to proteins, they can activate chemical pathways. The reversibility aspect of ligands enables it to act as a messenger—neither the target protein nor the ligand itself is permanently changed.
The path to obtaining this biochemical map is through imaging, which historically has been a challenge given high-resolution electron microscopes damage living cells. Specifically, the strong electron beams and vacuum conditions of transmission electron microscopes (TEMs) destroy biomolecules. To solve this, scientists developed cryo-electron microscopy in the 1970s, a method that uses frozen specimens under less intense electron beams. In the years to follow, electron microscopy for biomolecules achieved a number of improvements.
During the mid-'70s to the mid-'80s, Joachim Frank at Columbia University in New York developed the technique of creating high-resolution three-dimensional structures from the processing and merging of lower resolution two-dimensional images. Jacques Dubochet at the University of Lausanne in Switzerland, figured out how to enable biomolecules to retain their shape in a vacuum by vitrifying water in the early ‘80s by rapid cooling water around the biological specimen. In 1990, Richard Henderson at the MRC Laboratory of Molecular Biology in Cambridge, United Kingdom, achieved atomic resolution of three-dimensional images of proteins using an electron microscope.
Together, Dubochet, Frank, and Henderson were equally awarded the Nobel Prize in Chemistry in 2017 for contributing to cool microscope technology (literally and figuratively) that enables researchers to see biochemical processes at atomic resolution and produce three-dimensional structures of biomolecules.
Having atomic resolution of the three-dimensional structure of biomolecules is only one piece of the drug discovery equation. Next comes the hard part of making sense out of these high-resolution images—a time-consuming and complex task for the human eye. This is where artificial intelligence machine learning can help.
For this study, the Japanese research team of Yasushi Okuno, Kei Terayama, Shigeyuki Matsumoto, Shoichi Ishida, Mitsugu Araki, and Takayuki Kato, used deep learning to create dynamics extraction from cryo-EM map (DEFMap)–a three-dimensional convolutional neural network (CNN). The researchers trained DEFMap on 25 cryo-EM maps from the Electron Microscopy Data Bank (EMDB), then tested it using three more cryo-EM maps.
“By relying only on cryo-EM maps, DEFMap successfully provided dynamics information equivalent to that determined from molecular dynamics (MD) simulations and experimental approaches at the atomic and residue levels,” the researchers wrote. “Additionally, DEFMap could detect dynamics changes associated with molecular recognition and the accompanying allosteric conformational stabilizations, which trigger various biological events such as signal transduction and enzyme catalysis.”
Now with this proof-of-concept, researchers have demonstrated that applying artificial intelligence (AI) machine learning can draw out meaningful patterns from cryo-EM protein images—a deep learning tool with the potential to accelerate drug discovery in the future.
Copyright © 2021 Cami Rosso All rights reserved.