Artificial Intelligence
Can an “AI Physicist” Outperform Einstein?
MIT’s AI system is able to derive laws of physics in mystery worlds.
Posted December 20, 2018
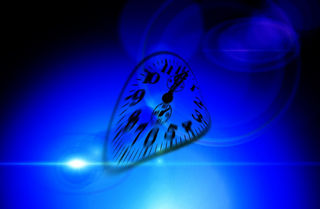
Physics is an important scientific field—it is a fundamental science with principles that impacts other branches of natural sciences. It explains the nature and properties of matter, energy, motion and force. The laws of physics have real-world relevance, and are deployed into products and services used in everyday modern living. Ranging from cars, airplanes, smartphones, headphones, to see-saws in a playground, the laws of physics impact the way we live. Recently a research team from the Massachusetts Institute of Technology (MIT) created an artificial intelligence (AI) system that deploys centuries old heuristics of physicists to derive laws of physics from complex, simulated worlds. Can a machine learning physicist understand the natural laws of our world better than a human physicist?
Applied physics has brought innovation in optics, energy, electronics, lasers, lidar, computing, magnetic resonance imaging, sonar, radar, semiconductors, materials science, electromagnetic propulsion, and space exploration. Interdisciplinary physics include neurophysics, psychophysics, acoustics, nanotechnology, biophysics, astrophysics, and econophysics. Breakthroughs in physics has real-world commercial opportunities.
The MIT research team of Tailin Wu and Max Tegmark incorporated four strategies that are commonly-used by physicists: divide-and-conquer, Occam’s razor, unification, and lifelong learning in developing their algorithm for the AI Physicist.
Physicists throughout the ages have provided us theories to explain natural phenomenon and our universe such as quantum mechanics, general relativity, Newton’s law of universal gravitation, Big Bang, M-theory, superstring theory, grand unification theory, BCS theory, and Bloch theory, to name a few. Wu and Tegmark used an innovative approach that is centered on the machine learning and processing of theories, rather than the standard method of applying a single large model to all of the data.
Wu and Tegmark deployed a divide-and-conquer strategy to find individual theories from complex observations. The algorithm learns multiple theories that describes part of the overall data. Each theory are encouraged algorithmically to specialize in its respective domain by rewarding larger gradients for better performing theories.
Occam’s razor, also known as the law of parsimony, is a problem-solving approach that when giving multiple hypotheses, the one that has the least amount of assumptions is more likely to be correct. In other words, the simpler the explanation, the better. Occam’s razor was incorporated by minimizing the total description length mathematically in the algorithm. Specifically, the method used Solomonoff’s theory of inference linked to Hutter’s AIXI approach to artificial general intelligence.
By using the strategic concept of unification, Wu and Tegmark introduced specific parameters with the goal of unifying learned theories. It’s about identifying fundamental similarities between various theories, and bringing them together in one comprehensive theory. The desired goal is to have a master theory that can generate a continuum of theories.
The team embedded the concept of lifelong learning into the architecture so that knowledge is accumulated and past experiences are preserved. The model remembers learned solutions and tests them on future problems.
The resultant unsupervised machine learning agent, named “AI Physicist,” was tested in increasingly complex physics environments in simulated worlds that had random combinations of harmonic motion, elastic bounces, gravity, and electromagnetism.
The team created 40 “mystery” worlds with natural laws of physics that varied from one location to another. In the experiment, in addition to testing the AI Physicist, Wu and Tegmark had a “baseline” agent, and a “newborn” AI Physicist that has not been exposed to past examples, and therefore a lifelong learning strategy had no impact. Both the Newborn and AI Physicist showed capabilities of solving above 90 percent of all the 40 mystery worlds. The team discovered that just like with human scientists, a lifelong learning strategy helps the AI Physicist to perform better and learn faster with less data in new environments.
Out of all of the scientific fields, physics is arguably one of the best ones to apply artificial intelligence. The universe is inherently complex, and harnessing the pattern-recognition capabilities of unsupervised machine learning could potentially reveal some novel insights. Wu and Tegmark seek to “improve techniques for simplifying complex learned neural nets.” They plan to further reduce and simplify AI Physicist’s future model architecture to improve both the speed and accuracy of future unsupervised machine learning agents.
According to the researchers, AI Physicist “typically learns faster and produces mean-squared prediction errors about a billion times smaller than a standard feedforward neural net of comparable complexity.”
Can AI Physicist eventually evolve to understand the natural laws of our world better than a human physicist? Watch this space over time.
Copyright © 2018 Cami Rosso All rights reserved.
References
Wu, Tailin, Tegmark, Max. “Toward an AI Physicist for Unsupervised Learning.” arXiv:1810.10525. November 6, 2018. Retrieved 12-19-2018 from https://arxiv.org/pdf/1810.10525.pdf