Bias
Have We Been Ignoring the Biggest Flaw in Human Judgment?
A new book by Daniel Kahneman and colleagues on the silent problem of noise.
Posted August 21, 2021 Reviewed by Vanessa Lancaster
Key points
- Judges, doctors, personnel committees, and stock portfolio managers make wildly different decisions based on the same evidence.
- Kahneman, Sibony, and Sunstein make a strong case that such noisy judgments are common, and lead to as many bad decisions as do cognitive biases.
- Noisy judgments are costly, unfair, and sometimes downright dangerous
- Kahneman and colleagues offer handy suggestions for reducing costly noise in human judgment.
Daniel Kahneman won a Nobel Prize for his work on biases in human judgment. Those judgmental biases are not just relevant to intellectual nerds interested in dissecting cognitive processes. They matter in everyday life for all of us.
If your nephew is convicted of drug possession while on vacation, he may confront a judge who has especially harsh attitudes about drugs and end up having the book thrown at him; or he might luck into an easygoing judge who lets him off with a warning.
Courtroom judges aren't the only people who exercise judgmental biases. Businesspeople make judgments about whether a particular candidate is more qualified for a job than the other candidates. University faculty make judgments about accepting or rejecting graduate school applicants, bankers judge who will get a loan at what interest rate. Physicians judge how to diagnose a particular person’s symptoms and which course of treatment to prescribe.
Kahneman became an academic rock star because he demonstrated several systematic biases in judgment, such as the availability heuristic (we often overestimate the prevalence of things that come readily to mind). But in a new book, he joins Olivier Sibony and Cass Sunstein to argue that judgmental biases, although they have received an immense amount of press, are only a part of the problem (Kahneman, Sibony, and Sunstein, 2021).
The other huge part of the problem has been largely ignored – judgments are often very noisy. Ask ten different judges to suggest a sentence for your nephew, and you are likely to get widely divergent answers, as demonstrated in research by legal scholars. This is, of course, unfair.
Ask ten different doctors to diagnose a patient with a complicated set of presenting symptoms, and you may well get widely divergent diagnoses. This is not so much unfair as potentially dangerous. Physicians often misdiagnose, which leads to mistreatment that can sometimes lead to death.
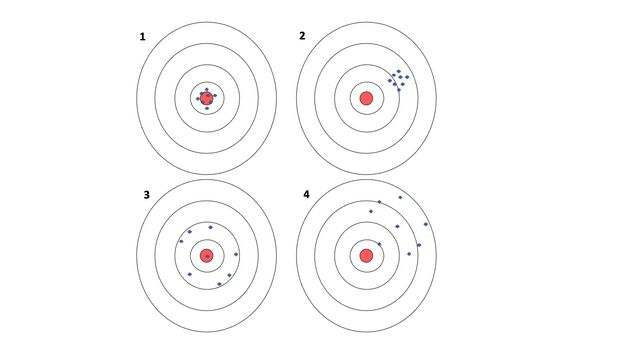
Kahneman et al. presented a useful mnemonic device for distinguishing between bias and noise. Imagine four different targets that have each been shot at by eight different gunslingers. One target (see Figure, upper left) has eight holes closely clustered near the bullseye – this demonstrates low bias and low noise. A second target has eight holes clustered around ten inches to the upper right of the bullseye – this demonstrates high bias but low noise. A third has eight holes widely dispersed around the bullseye, showing low bias but high noise. The fourth target has eight widely distributed holes, all leaning to the upper right, demonstrating noise and bias.
The authors noted several different sources of noise in human judgment –one problem is individual differences (in the tendency to judge targets harshly or benignly, for example, which is called level noise). Besides level noise, another important source of error is pattern noise - one judge may be harsh in his penalties for drunken driving, but relatively benign in his punishments for tax evasion; whereas another judge may show the reverse pattern (as Kahneman notes, this can also be called the judge x problem interaction). Another is the use of judgment scales that use vague and imprecise subjective descriptors. Still another is random variation in the order in which judges see different sources of evidence.
Kahneman et al. also suggested several helpful hints about how to reduce noise. One is to have all judges apply a strict set of decision rules, with no room for subjective judgment (research has consistently demonstrated that computational formulas outperform even highly expert individual judges). Another is to have different members of a team judge different dimensions separately (in accepting graduate students, one person evaluates their research experience, another evaluates the quality of letters, another evaluates their grades and test scores, for example). Judges should not talk to one another during the evaluation process but record their conclusions before any discussion. Finally, the authors suggested making relative judgments and using ranking (in judging job applicants, or evaluating employees, for example, rank them all against one another rather than using a scale that allows everyone to be rated as “excellent”).
Kahneman et al. presented several real-life cases demonstrating the prevalence and importance of noise and addressing some of the common objections to reducing noise. One of the most troubling cases involved judicial penalties for the same crime. Judges like to think that they are tailoring the punishment to each particular case.
However, evidence suggests that different judges judging the same criminal case present wildly different sentences. And the same judge may be harsh or lenient depending on what time of the day they are making the decision.
After the evidence about noise in sentencing became widely known, there was an attempt to apply fairer rules by developing standardized penalties for the same crime. Unfortunately, judges rebelled, the corrective laws were overturned, and the unfair variation in the distribution of penalties returned.
Noise in human judgment is a serious problem, and it results in a great deal of inefficiency and unfairness. Hence, this ought to be required reading for anyone who ever makes judgments with implications for their future or for the lives of others, which is pretty much every one of us (in my judgment).
References
Kahneman, D., Sibony, O., & Sunstein, C. (2021). Noise; A flaw in human judgment. New York: Little Brown.