Artificial Intelligence
Can Artifical Intelligence Be Conscious?
Testing for AI consciousness, and what AI consciousness means for society.
Updated September 6, 2024 Reviewed by Monica Vilhauer Ph.D.
Key points
- Artifical intellignece may be conscious one day.
- Developing a consciousness test is difficult.
- Quantum mechanics may provide a soluton for testing AI consciousness via an observer-centric ontology.
- Physicist John Wheeler's participatory universe and QBism via N-Frame may be key.
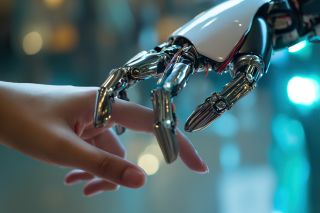
In the last few years, there has been an explosion of rapid advances in artificial intelligence (AI), particularly in AI’s ability to engage in conversational style text (called natural language processing; NLP) such as Open AI’s ChatGPT, Google’s Gemini, and Anthropic’s Claude models. This sudden increase in ability is due to recent developments in transformer architecture, which started with the release of Google’s seminal paper called “Attention is All You Need” in 2017 [1].
The transformer architecture is a type of deep learning neural network, with the key innovation being the “attention mechanism,” which allows it to weigh the importance of different parts of the input data (of some text conversation) dynamically. The model starts by converting input data (such as text) into embeddings, which are numerical representations of words or tokens. These embeddings capture semantic information and context about the words. Since transformers do not have a built-in notion of the order of words, positional encodings are added to the embeddings to provide information about the position of each word in a sentence. This helps the model understand the sequence of the input data.
The core of the transformer model is the attention mechanism, specifically "self-attention." Self-attention allows the model to focus on different parts of the input sequence when producing an output. It computes a weighted sum of input values, where the weights are determined by how relevant different words are to each other. In practical terms, for each word in a sentence, self-attention calculates attention scores with every other word. These scores are then used to create a weighted combination of all words to produce a representation for each word that considers its context. The transformer uses multiple self-attention mechanisms, called "multi-head attention." This allows the model to capture different types of relationships and dependencies between words from different perspectives or "heads." After the attention layer, each word representation is passed through a feed-forward neural network, which further processes the information and captures complex patterns. The model uses also layer normalization and residual connections to stabilize and speed up training. Residual connections help in preventing the degradation of performance as the network becomes deeper. Multiple layers of attention and feed-forward networks are stacked on top of each other. Each layer allows the model to learn increasingly complex representations and patterns. For tasks like language translation or text generation, a decoder part of the transformer architecture generates the output sequence, one word at a time, using the encoded input representations and previously generated words.
Transformers have been incredibly successful in a variety of NLP tasks, such as language translation, text summarization, question answering, and sentiment analysis. Transformer architecture represents a significant leap in AI, especially in the field of NLP, due to its efficiency and ability to understand complex patterns in data. Their innovations include advantages in (1) parallelization, where, unlike previous models such as RNNs, which process sequences one step at a time, transformers can process entire sequences in parallel, making them much faster to train; (2) scalability, whereby transformers are highly scalable and can handle very large datasets, making them suitable for training with large amounts of data; (3) flexibility, whereby the attention mechanism allows transformers to model long-range dependencies and complex relationships in data.
Given these innovations, several philosophers and researchers have asked whether AI can achieve human-level intelligence called artificial general intelligence (AGI), and even become conscious. Alan Turing in 1950 devised one of the first AI tests for intelligence called the imitation game, more commonly known as the Turing Test [2]. The test is designed to assess a machine's ability to exhibit intelligent behavior that is indistinguishable from that of a human. A machine is said to have passed the Turing Test if the human judge is unable to reliably distinguish between the human and the machine based solely on their conversational responses. In other words, if the machine's responses are indistinguishable from those of a human to the extent that the judge cannot tell them apart, the machine is considered to have demonstrated a form of human-level artificial intelligence. The problem with this test is that it is an intelligence test, not a consciousness test.
Philosopher and cognitive scientist David Chalmers [3] referred to the easy problem of consciousness as neural correlates of consciousness, and the hard problem of consciousness as finding an explanation of how physical neurons give rise to consciousness, such as the taste of chocolate or the feeling of compassionate love. In over 100 years of research, researchers are no closer to explaining how the brain produces a single conscious experience (qualia). Recent attempts to explain consciousness such as Orchestrated Reduction (Orch OR) [4], Integrative Information Theory (IIT) [5], and Global Workspace Theory (GTW) [6] all neglect to explain how a single conscious experience (qualia) arises.
My own recent work [7] suggests that the central solution to this problem lies in the ontological framework these models utilize. These are all physicalist (materialist) models based on a spacetime framework described in an Einsteinian Riemannian manifold. However, several breakthrough experimental findings in physics suggest that non-local realism (a physicalist Einsteinian realism based on a Riemannian manifold) is false at the fundamental level. This accumulated work led to the Nobel Prize awarded to Alain Aspect, John F. Clauser, and Anton Zeilinger for their experimental findings on Bell’s inequalities, first postulated by John Bell [8]. These findings consistently show violations of Bell’s inequalities, which imply that no local hidden variable theory can explain the quantum correlations observed in experiments. The violation of Bell's inequalities indicates that either:
- Information somehow travels faster than light, meaning distant events can instantaneously influence each other (non-locality).
- Or that we must abandon assumptions about local realism, i.e., the idea that objects have definite properties independent of measurement must be false, and quantum mechanics represents a reality that is more fundamentally probabilistic and context-dependent.
Accepting (1) non-locality means that communication can exist faster than light speed (which is assumed in general relativity to be constant), therefore Einstein’s local realism is false. This contradicts Einstein’s General Relativity, and therefore cannot logically be accepted without rejecting General Relativity as a complete and fundamental theory of the universe. Accepting (2) means that particles do not have well-defined properties (such as position or momentum) until they are measured. This runs counter to Einstein’s belief that objects such as the moon are still there when no one is looking at them. Instead, objects are rendered in real-time when looked at (observed). These quantum mechanical findings force us to accept that the properties of our universe may be indeterminate or only potential until observed, which falsifies any notion that we live in a fundamentally determined physicalist (material) universe.
These findings have led to some profound conclusions about our ontological reality, i.e., local physicalism is not our fundamental reality and only becomes experienced as "real" when observed. Consistent with these findings, my own recent research proposes a context-dependent observer-centric universe ontology via a functional contextual, evolutionary, and predictive coding behavioral model called N-Frame [9], that utilizes Quantum Bayesianism (QBism) to model quantum effects as epistemic conscious events. This is consistent with John Wheeler’s participator reality, whereby the observer is a participator to reality (the collapse or actualizer of the waveform – i.e., the physical reality we see as in interface) rather than passive. John von Newmann and Eugene Wigner held similar beliefs about a human conscious observer being responsible for the collapse of the waveform. N-Frame suggests that the physical reality is illusionary to something deeper (i.e., the quantum world), and acts to encode fitness to promote the survival and evolution of our underlying conscious agency.
This novel observer-centric universe ontology is consistent with experimental data not just of Bell’s inequalities but also of the quantum eraser effect, and recent physics experiments (called a double-slit experiment) which show humans collapsing (or actualizing) the quantum waveform via their conscious mental awareness [10]. Crucially these findings have a 5-sigma, that is a 1 in 3.5 million probability that the findings could be due to chance factors (similar to that of finding the Higgs Boson as CERN which led to a Nobel Prize). The recent work suggests that as a human can collapse the wave function non-locally, consistent with violations in Bell’s inequality (i.e., that quantum entanglement exists non-locally), this bi-passes problems of the hard problem of consciousness. The hard problem of consciousness is only hard when adopting a physicalist ontology of the universe, and when doing so becomes an impossible problem for physicalism. The hard problem of consciousness or matter (physicalism or materialism), simply disappears when assuming an observer-centric reality.
My own recent work [7] suggests that when adopting an observer-centric reality, then a consciousness causes (or actualizes) collapse quantum double slit experiment could be used to test AI consciousness, similar to those conducted on humans [10]. Finding a positive result for such experiments on AI (this would be perhaps a neuromorphic AI with some quantum computation to evoke entanglement), would essentially determine that the AI is indeed conscious. This could have great implications as to what this means for AI rights and whether they should be regarded as sentient. Currently, no AI collapse (or actualization) of the waveform has been observed in AI, but for the future only time will tell.
References
[1] Vaswani, A., Shazeer, N., Parmar, N., Uszkoreit, J., Jones, L., Gomez, A. N., et al. (2017). Attention is all you need. Adv. Neural Inf. Proces. Syst. 30. doi: 10.48550/arXiv.1706.03762
[2] Turing, A. M. (1950). Computer machinery and intelligence. Mind 59, 433–460.
[3] Chalmers, D. (2017). The hard problem of consciousness. The Blackwell companion to consciousness, 32-42.
[4] Hameroff, S., & Penrose, R. (2014). Consciousness in the universe: A review of the ‘Orch OR’theory. Physics of life reviews, 11(1), 39-78.
[5] Tononi, G. (2012). The integrated information theory of consciousness: an updated account. Archives italiennes de biologie, 150(2/3), 56-90.
[6] Baars, B. J. (2005). Global workspace theory of consciousness: toward a cognitive neuroscience of human experience. Progress in brain research, 150, 45-53.
[7] Edwards, D. J. (2024). A functional contextual, observer-centric, quantum mechanical, and neuro-symbolic approach to solving the alignment problem of artificial general intelligence: safe AI through intersecting computational psychological neuroscience and LLM architecture for emergent theory of mind. Frontiers in computational neuroscience, 18, 1395901.
[8] Bell, J. S. (1964). On the Einstein Podolsky Rosen paradox. Physics Physique Fizika, 1(3), 195.
[9] Edwards, D. J. (2023). Functional contextual implementation of an evolutionary, entropy-based, and embodied free energy framework: Utilizing Lagrangian mechanics and evolutionary game theory’s truth vs. fitness test of the veridicality of phenomenological experience. Frontiers in Psychology, 14, 1150743.
[10] Radin, D., & Delorme, A. (2022). Psychophysical Effects on an Interference Pattern in a Double-Slit Optical System: An Exploratory Analysis of Variance. Journal of Anomalous Experience and Cognition, 2(2), 362-388.