Cognition
How Do Computers and Humans Process Language?
Language structures yield good human and computational language models.
Updated March 13, 2024 Reviewed by Tyler Woods
Key points
- Humans acquire language with very limited training data, whereas ChatGPT requires massive amounts data.
- What are the differences between humans and computers when it comes to natural language processing?
- ChatGPT is argued to be trained on unstructured data, but is that really so, given the way humans are trained?
- Human and computational models of language processing perform so well because language is structured.
The performance of natural language models such as ChatGPT is deeply impressive. The performance of another one of these models, the one called "human,"is at least as impressive.
The human model stores only about 1.5 megabytes of data for a native language [1]. With relatively little training data, the human model is able to capture sentence structures, bootstrap the meaning of words, and generate words and language it has never seen or heard before. The human model does not really need to be told what is correct language use and what is not; it is able to learn this automatically. Basically, it is able to identify itself what is correct in terms of grammar, meaning, and context. It excels at language processing.
For the computational model (take ChatGPT as the example), whether you ask the model a knowledge question, to make a summary for you, or have it create an original limerick, poem, or haiku, it demonstrates human-like performance. One can argue that it perhaps even surpasses human performance. Whereas it might take you, as a reader, half an hour to come up with an original limerick for this blog post, it would take ChatGPT just a matter of seconds.
GPT stands for General Pretrained Transformers, artificial neural networks trained on massive amounts of data. Around 570GB of data are used to train a model consisting of about 1.8 trillion parameters across 120 layers. This training is extremely expensive both in terms of computing power and the resulting carbon footprint [2].
The human natural language model and the natural language model called "ChatGPT" show remarkable similarities in their output: generated language. For the input, the similarities between the two are obvious: both are trained on language data in order to generate language output.
And yet the computational and human language processing models also show remarkable differences. The amount of language data the computational model needs to be trained is very different than the amount of training data the human model needs. The computational power needed for the computational model is also very different from the power the human model needs. How can we explain these similarities and differences?
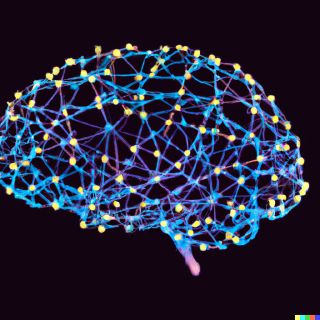
Unstructured data?
It is often argued that artificial intelligence models like ChatGPT are trained on unstructured data. If an AI model recognizes an image, the pixels in the image are "unstructured." The language data AI models like ChatGPT are trained on could be considered equally unstructured. This is true to some extent. Indeed, pixels in images, just like words in sentences, are not tabularized in a structured spreadsheet, but is the input really unstructured?
Think about it. If we were to take a 10x10 image (100 pixels) with 256 grayscale levels, we could create 6.668E+240 different images. That’s a lot of images! So many images that if we assume an average person lives to 90 years old, we would need to see 2.3493E+231 pictures per second throughout our lifetime to have seen all pictures. Now, computers might process faster depending on computational resources, but 6.668E+240 different images—and only the ones that are 100 pixels—is even a lot for computers.
For language, things are not much different. Let’s take a single word. If a word were truly unstructured, all letter combinations could exist. If we take words with maximally 10 letters, we can then create 1.46814E+14 different words. Mind you, the word “unstructured” would not exist in this set of words, because it has 12 and not 10 letters. If we assume 171,476 words in the Oxford English Dictionary, there are 51,727 times more 10-letter words that we can form than all the words that actually exist in the dictionary. I have argued elsewhere that we have no problem recognizing 10 sextillion sentences—as many sentences as there are grains of sand in the Sahara desert—which makes it rather difficult to argue that language models are trained on unstructured data [3].
Perhaps the magic of the computational and human natural language models does not lie in training data and computational power. Perhaps the magic lies elsewhere in the language system itself. With an increasing amount of evidence that language is not arbitrary—the sound of words gives pieces of meaning away, as does word order, as does the context of words—what human and computational models are actually doing is picking up on these language patterns.
That would be an exciting conclusion, as it would explain how humans are so good at language and why computers can become so good at language (and how the latter might actually decrease their carbon footprint by taking advantage of these patterns in language).
In closing, I guess I owe you the limerick I mentioned earlier. Here is the one created by ChatGPT:
In the land where language takes flight,
Both human and AI shine bright.
From pixels and word,
Meaning's melody's heard,
In patterns, they find their delight.
References
[1] Mollica, F., & Piantadosi, S. T. (2019). Humans store about 1.5 megabytes of information during language acquisition. Royal Society Open Science, 6(3), 181393.
[3] Louwerse, M. (2021). Keeping those words in mind: How language creates meaning. Rowman & Littlefield.