Artificial Intelligence
AI and VR Transform Thoughts to Action with Wireless BCI
New wireless BCI uses AI and virtual reality to convert brain imagery to action.
Posted July 26, 2021 Reviewed by Kaja Perina
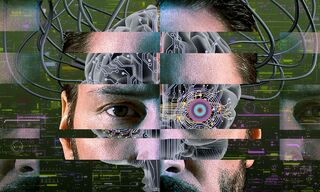
The aim of brain-computer interfaces (BCIs), also called brain-machine interfaces (BMIs), is to improve the quality of life and restore capabilities to those who are physically disabled. Last week, researchers at the Georgia Institute of Technology and their global collaborators published a new study in Advanced Science that shows a wireless brain-computer interface that uses virtual reality (VR) and artificial intelligence (AI) deep learning to convert brain imagery into actions.
The brain-computer interface industry is expected to reach USD 3.7 billion by 2027 with a compound annual growth rate of 15.5 percent during 2020-2027 according to Grandview Research.
“Motor imagery offers an excellent opportunity as a stimulus-free paradigm for brain–machine interfaces,” wrote Woon-Hong Yeo at the Georgia Institute of Technology whose laboratory led the study in collaboration with the University of Kent in the United Kingdom and Yonsei University in the Republic of Korea.
The AI, VR with BCI system was assessed on four able-bodied human participants according to a statement released on Tuesday by the Georgia Institute of Technology.
Brain-computer interfaces assists those who are physically disabled due to due locked-in syndrome, brain injuries, paralysis, or other diseases and disorders that impact motor function. Over 131 million people globally use a wheelchair according to estimates from the Wheelchair Foundation, and in the United States, an estimated 5.4 million people are living with paralysis according to the Christopher & Dana Reeve Foundation.
“Conventional electroencephalography (EEG) for motor imagery requires a hair cap with multiple wired electrodes and messy gels, causing motion artifacts,” the team of scientists wrote.
In contrast, the new BCI offers a portable, low-profile scalp electronic system with virtual reality (VR), wireless circuits and microneedle electrodes.
“For mobile systems, dry electrodes are preferred due to short setup times, no skin irritation, and excellent long-term performance,” the researchers wrote. “In addition, they often perform better than gel-based EEG sensors while providing long-term wearability with-out reduced signal quality.”
According to the scientists, virtual reality provides consistent visuals, as well as clear and instant biofeedback when handling subject variance in detectable EEG response to motor imagery.
“The wearable soft system offers advantageous contact surface area and reduced electrode impedance density, resulting in significantly enhanced EEG signals and classification accuracy.”
The scientists applied artificial intelligence machine learning to the time-domain data generated. Specifically, they used a convolutional neural network (CNN) model for the preprocessing and classification of the motor imagery brain signals. AI deep learning was used to decompose spatial features from multiple dipolar sources located in the motor cortex of the brain.
Convolutional neural networks are a common type of deep learning neural networks that are well-suited for image classification and object recognition tasks. Artificial neural networks are a subset of machine learning made of an input layer, one or more hidden layers, and an output layer. CNNs are modeled after the biological brain with multiple layers of nodes that act like artificial neurons. The first layer of the network is a convolutional layer, followed by pooling layers, with a fully connected (FC) layer as the final layer. Each layer has interconnected nodes that act like artificial neurons with an associated weight and threshold. When the output of a single node is greater than the threshold value, the node is activated, and data is passed to the next layer of the network.
CNNs require less preprocessing of the images. A convolution is a mathematical operation on two functions that produce a third function that expresses how the shape of one is changed by the other.
“The combination with convolutional neural network-machine learning provides a real-time, continuous motor imagery-based brain–machine interface,” concluded the researchers. “With four human subjects, the scalp electronic system offers a high classification accuracy (93.22 ± 1.33% for four classes), allowing wireless, real-time control of a virtual reality game.”
Georgia Institute of Technology has a patent-pending for the wireless brain-computer interface.
Copyright © 2021 Cami Rosso All rights reserved.