Artificial Intelligence
Why ChatGPT Talks the Talk but Doesn’t Walk the Walk
Are large language models as intelligent as humans?
Updated October 11, 2023 Reviewed by Ray Parker
Key points
- Many wonder how the intelligence of large language models compares to human intelligence.
- Humans have an advantage in learning speed and flexibility in applying what they have learned.
- Humans remain unique in their ability to respond relationally, the foundation of intelligent behavior.
By Matthias Ramaekers
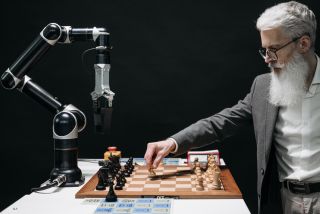
You have probably noticed that artificial intelligence (AI) research is a booming business. Recent advances in large language models (LLMs), like the one powering ChatGPT, surprised the world.
Their capacity for humanlike language and problem-solving abilities led many to imagine potential applications or risks to society—including Geoffrey Hinton, the "godfather of AI."
Others pointed out that LLMs have comparatively limited capacity for activities that humans perform with little effort, such as humor [1], causal and counterfactual reasoning [2], and logic [3]. So, to what extent do these models truly mimic human, or indeed general, intelligence?
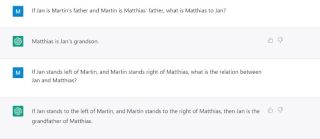
Answering that question requires a definition of intelligence, but there is a lot of debate about how to define intelligence, whether human or artificial intelligence [4-5]. Leaving these debates to the side, I'll focus on a characteristic of intelligent systems that my colleagues in the Learning and Implicit Processes Lab are interested in: the ability to learn, that is, to change behavior as a function of past experiences [6].
Humans and many other organisms are capable of learning [7]. Many learning psychologists like myself would be out of a job if they were not. LLMs like that under the hood of ChatGPT also learn from experiences using various learning algorithms.
How do we compare these systems? We can differentiate them in terms of the speed and efficiency with which they learn and the flexibility and creativity by which they apply learned knowledge. We now see significant differences between humans, other animals, and artificial systems.
In terms of speed, humans have a clear advantage over state-of-the-art AI. In short, LLMs are trained on massive amounts of data, from which they essentially learn to predict which (parts of) words are most likely to follow previous (parts of) words, followed by a second round of training to finetune their responses to human-produced prompts and questions.
This learning history is sufficient to produce remarkably humanlike responses. Still, LLMs typically require about four to five orders of magnitude, or roughly 50,000 times more training instances than the average human child, to produce that behavior [8]. ChatGPT's training is a rather brute-force approach to learning to produce intelligent linguistic behavior. It allows it to pick up on the underlying regularities of human language, but it differs significantly from how humans acquire language.
Humans are not only faster but also more flexible learners. Children quickly acquire the ability to reason about novel problems flexibly and to adapt to new situations after only a few exposures or mere instruction.
This feat remains a challenge for state-of-the-art AI. LLMs have much-improved generalization capacity, but it is hard to systematically assess because their training encompasses virtually everything humans have ever written. When probed on novel problems or instances of familiar problems, you can see the limits of its abilities, suggesting its responses reflect something more similar to memorizing problem solutions than thorough understanding.
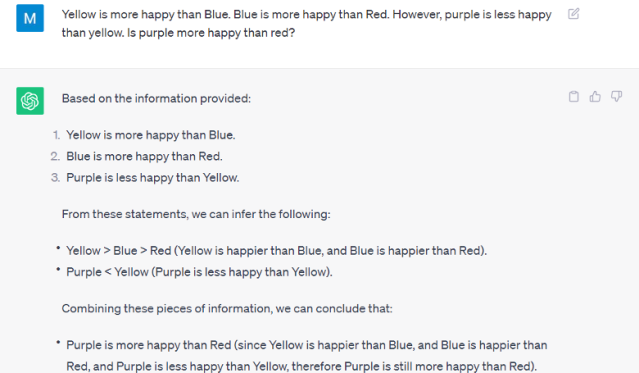
Current LLMs are not an ideal model for learning psychologists investigating how humans learn. What, then, makes humans so unique?
In our lab, we take the perspective of relational frame theory (RFT) [9], which proposes that human cognition, and thus intelligence, is founded on our ability for relational responding—responding to one event in terms of its relationship to another.
As we grow up in a verbal, social community, we learn to relate objects and events in our environment in many ways (A is the same as B, A is more than B, A is less than B, A is part of B, A comes after B, A is here and B is there, A is to B as C is to D, and so on). We also learn to respond to those relations appropriately (e.g., if box A has one chocolate, and box B has more than box A, which do you choose?). Over time, the relations can be abstracted away from the irrelevant physical properties of the related objects, and we can start applying them to objects arbitrarily or symbolically (e.g., responding to the word "dog" in the same way you would to an actual dog).
This ability to "act as if" things are related provides the foundation for fast, flexible, and generative learning and, ultimately, what we consider to be intelligent behavior (see [10] for more examples). It is no coincidence that many of the subtests that make up modern intelligence tests (e.g., Raven's Progressive Matrices and other fluid reasoning tests, verbal reasoning, visuospatial reasoning, etc.) assess behavior that is relational and that there is preliminary evidence suggesting relational training can help increase children's intelligence [11]
Of course, many in AI acknowledge the importance of relational reasoning and have attempted to incorporate relational mechanisms into their models. So far, however, none seem to have reached the "symbolic level" still uniquely occupied by humans. Suppose AI research wants to mirror the processes underpinning human abilities and not just produce humanlike output as it does now.
In that case, it will require a different approach focused on efficient, context-sensitive learning. In my search in the LIP lab, we try to do this by developing computational models inspired by the core ideas of RFT, which, if the theory is correct, should provide a better (i.e., faster and more flexible) model of human learning.
Returning to the question of ChatGPT's intelligence, it is important to note that it was developed with a specific purpose: to interact with humans through a computer interface and produce coherent answers to whatever prompt it gets. With that goal in mind, its performance is remarkable.
It was not designed to be generally intelligent (i.e., capable of flexibly adapting to novel situations or problems), and it isn't. Still, it gives us the illusion of intelligence because it mimics intelligent human language.
Matthias Ramaekers is a researcher at the Learning and Implicit Processes Lab. The author wishes to thank Jan De Houwer and Martin Finn for their feedback on earlier versions.
References
For more, read this clarifying piece by mathematician Stephen Wolfram.
[1] Jentzsch, S., & Kersting, K. (2023). ChatGPT is fun, but it is not funny! Humor is still challenging Large Language Models. arXiv preprint arXiv:2306.04563.
[2] Chomsky, N., Roberts, I., & Watumull, J. (2023). Noam Chomsky: The False Promise of ChatGPT. The New York Times, 8.
[3] Liu, H., Ning, R., Teng, Z., Liu, J., Zhou, Q., & Zhang, Y. (2023). Evaluating the logical reasoning ability of chatgpt and gpt-4. arXiv preprint arXiv:2304.03439.
[4] Sternberg, R. J. (2018). Theories of intelligence. In S. I. Pfeiffer, E. Shaunessy-Dedrick, & M. Foley-Nicpon (Eds.), APA handbook of giftedness and talent (pp. 145–161). American Psychological Association. https://doi.org/10.1037/0000038-010
[5] Wang, P. (2007). The logic of intelligence. In Artificial general intelligence (pp. 31-62). Berlin, Heidelberg: Springer Berlin Heidelberg.
[6] De Houwer, J., Barnes-Holmes, D., & Moors, A. (2013). What is learning? On the nature and merits of a functional definition of learning. Psychonomic Bulletin & Review, 20, 631-642.
[7] De Houwer, J., & Hughes, S. (2023). Learning in individual organisms, genes, machines, and groups: A new way of defining and relating learning in different systems. Perspectives on Psychological Science, 18(3), 649-663.
[8] Frank, M. C. (2023). Bridging the data gap between children and large language models. Trends in Cognitive Sciences.
[9] Hayes, S. C., Barnes-Holmes, D., & Roche, B. (Eds.). (2001). Relational frame theory: A post-Skinnerian account of human language and cognition.
[10] Hughes, S., & Barnes‐Holmes, D. (2016). Relational frame theory: The basic account. The Wiley handbook of contextual behavioral science, 129-178.
[11] Cassidy, S., Roche, B., Colbert, D., Stewart, I., & Grey, I. M. (2016). A relational frame skills training intervention to increase general intelligence and scholastic aptitude. Learning and Individual Differences, 47, 222–235. https://doi.org/10.1016/j.lindif.2016.03.001