Cognition
What Psychologists Still Haven’t Learned From Moneyball
Analytics changed sportswriters' thinking, but some psychologists lag behind.
Posted May 11, 2019
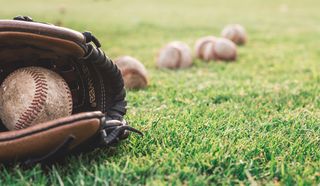
As an uncoordinated nerd in high school, the book Moneyball by Michael Lewis was my primary entry point for fantasizing about sports.
In the book, heroic front-office employees at a small market baseball team use their knowledge of statistics to create a winning baseball team against the odds. (In this case, their hardship was not having as many millions of dollars to spend on salaries as the Yankees.) Nerdy white-collar workers become sports legends through math!
Since then, analytics have turned baseball upside-down. Sportswriters and broadcasters drop complicated statistics into their daily patter about games, commenting on "Wins Above Replacement" and "On-base Plus Slugging."
One of the biggest changes I’ve seen in sports writing over that time is a broadening knowledge of one important aspect of statistics: small sample sizes.
In my memory, there were always articles in Spring Training and early on in the season about how a player was breaking out after one big week. Look! Josh Towers is the new Orioles ace!
These days, I always see articles about hot starts prefaced with a disclaimer about small sample sizes.
Sportswriters have learned: You can’t make firm conclusions about how good a player is by just looking at handful of games.
As heartening as it is to see the general statistical literacy in sportswriters going up, it’s been just as hard to realize that these insights are only slowly starting to trickle through to the scientific literature in psychology.
Psychologists use statistics, because we don’t expect people to behave in perfectly deterministic ways. There aren’t known equations that perfectly predict how well people will get along at a party, or how long you’ll be sad after a breakup. Statistics assume that by looking at lots of observations together and making the right comparisons, we can get a good picture of what will happen in general, to most people.
To get a good enough idea of what will happen, you need enough observations.
How many breakups would you need to observe before you’d be willing to make a confident statement about what breakups are like for most people? 10? 20? 100?
In part, it depends on what aspect of a breakup you’re talking about. If you’re talking about a big, general effect—will people feel sad?—then maybe you would feel comfortable after only seeing 20 or 30 (and comparing them to 20 or 30 people who didn’t break up).
If you’re talking about a small, specific effect—will people be more likely to learn an important personal lesson?—then maybe you’d want to see 100 breakups.
In general, though, you’d realize that research on small groups of people is limited. Like sportswriters now routinely do, you’d want scientists to include a disclaimer on these kinds of studies: Don’t take this too seriously, because we don’t have enough information to make a firm conclusion.
Just like you wouldn’t trust a sportswriter who said a player was going to be MVP, because he hit three home runs in one week, you also shouldn’t trust a scientist who claims that they’ve “found the brain region for comedy” if they have only looked at 20 brains.
The problem is that for decades psychologists were trained using rules of thumb about how many people they needed to include in a study—and these rules of thumb were horribly off the mark.
As a famous social psychologist wrote in a 2016 article:
“When I was in graduate school in the 1970s, n=10 was the norm, and people who went to n=20 were suspected of relying on flimsy effects and wasting precious research participants. Over the years, the norm crept up to about n=20.”
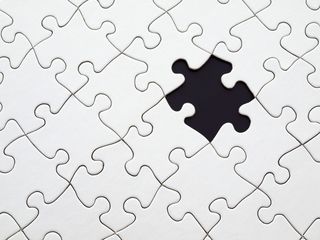
By “n=10” or “n=20”, the author means that the number of participants in each condition in a study would be 10 or 20 people.
This is far too low to detect anything but the most obvious effects. As Joe Simmons and colleagues presented in a 2013 talk, here are some effects that you can reliably detect with only 20 people in a condition:
- Men are, on average, taller than women.
- People above the median age report being closer to retirement.
Here are some effects that require more than 20 people in a condition to detect:
- People who like spicy food are more likely to like Indian food.
- Men, on average, weigh more than women.
Given the way that an earlier generation of social psychologists were trained, most of them were doing studies that weren’t able to reliably detect an effect more subtle than: “If you’re older than half of the people in the U.S., you’re planning on retiring sooner.”
They were essentially trained to do research the way journalists wrote about baseball before Moneyball. Any player who hit three home runs in a week was for sure the new MVP!
Only in the case of social psychology, it was more likely to be that 34 people told to avoid eating chocolate chip cookies spent more time working on an unsolvable puzzle—and so having to avoid cookies for sure made you less willing to try hard on a mental task, and so self-control was for sure a common resource that was drained by resisting temptation. And sometimes that finding would be so well-publicized the president would mention it in a speech.
What does this mean for you and me, trying to read psychology studies done in the 80s, 90s, and early 2000s?
To understand that, you would also need to know that researchers typically only try to publish studies that show “an effect”—a difference between groups.
Statisticians have shown through formal simulations that when you combine publication bias (a tendency to publish only results that show an effect) with sample sizes that are too small, you can get a published literature with effects that are 5 to 10 times too big—and often go in the wrong direction (e.g., breakups make you less sad!).
Knowing what we do about sample sizes, then, I always add the small sample size disclaimer when I read psychology research. Most studies that look like they have results that are “MVP-level exciting” are probably just an interesting blip until we get more information.