Decision-Making
Data Can Often Be Used to Tell Many Stories
We should be cognizant of how we transform data to answer the questions we do.
Posted December 8, 2022 Reviewed by Hara Estroff Marano
Key points
- Conclusions we draw are often affected by choices we make about which data to use and how we use them.
- Data choices we make are often affected by various biases and frames of reference we bring to the decision situation.
- Therefore, it is important to remember that all data are contextual rather than absolute.
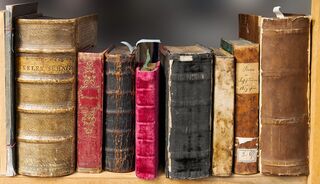
Most people think it’s more dangerous to live in a big city than it is to live in a suburban or rural area. After all, violent crime tends to be much higher in urban areas (Anderson, 2022), and this includes homicides. So, if your primary concern is to reduce the risk of death by homicide, steering clear of urban life is your best bet.
But that’s not the only way to quantify how dangerous different population centers are, as Fox (2022) recently showcased using data from 1999 to 2020[1]. When looking at only homicides, large metropolitan areas are more dangerous than nonmetro areas. But as soon as you factor in transportation accidents as a cause of death to accompany homicides, nonmetro areas become much more dangerous overall (roughly 80% more dangerous than New York City specifically and 35% more dangerous than large metropolitan areas more generally)[2].
The results change again when you broaden the cause of death to “external causes” (e.g., accidental poisoning, surgical complications). By this metric, nonmetro areas show 102% greater risk than New York City and 29% greater risk than large metropolitan areas more generally. So, is living in a big city really more dangerous than other population centers? The conclusion you reach will vary based on which risks you include.
I didn’t present the example above to advocate for people to move to large metropolitan areas[3]. My example was presented to highlight how the conclusions we draw are often affected by choices we make—from which data we use to how we aggregate it to specific analyses we conduct. And, at least in this example, data from the same source (Fox used CDC data) could be used to argue that large metropolitan areas are both more dangerous and less dangerous than nonmetro areas.
Lest you think this is an isolated incident, such an issue can occur in many other situations. For example, Fattal (2020) and Cartlidge (2021) both show, using very different types of research domains, that how analysts make decisions about their analysis process often determines the results they obtain. So, this isn’t an isolated issue.
The point I want to make from all this is that data or evidence is seldom truly objective in nature. A lot of human decision-making goes into which data to collect, how to aggregate and analyze it, and, subsequently, which conclusions to draw from it.
Let’s take the determination of which city is most dangerous as an example. I already showed how defining most dangerous based on homicides produces a very different conclusion than most dangerous when considering homicides and transportation fatalities. So, which is most dangerous is already open to subjective interpretation.
Dangerousness has to be operationalized (defined in a way that permits relative or absolute comparison among options) to be of any use for drawing conclusions, which will likely be influenced by the decision maker’s psychological context (the attitudes, beliefs, biases, and prior experience of the decision maker). Various biases are likely to come into play as a part of the psychological context, from motivational biases (what’s the reason for the decision) to values-based biases (the weight attached to different outcomes). These biases can alter the entire decision-making process.
For example, let’s presuppose a mayor of a large metropolitan area (Metro A) is going up for re-election soon. In this scenario, the aggregate of homicide and transportation-related deaths compares favorably to other comparable metropolitan areas (Metros B and C), showing lower aggregated risk in Metro A than in B and C. The mayor’s campaign might take this as evidence of the mayor’s success and conclude that the evidence supports the mayor’s re-election. After all, using this operationalization, Metro A is less dangerous than Metros B and C. But there is also an obvious incentive to stop at this message, as it portrays the mayor in a favorable light.
Let’s further presuppose, though, that the aggregate numbers are better overall for Metro A, but this is largely because of markedly lower risk from transportation-related deaths compared to B and C. It turns out homicide rates are much higher in Metro A than in B or C. The mayor’s opponent seizes on this information to argue the exact opposite conclusion, that Metro A is more dangerous than B or C and points to the homicide rate differences as evidence of the need for a mayoral change.
Technically, due to differences in how they operationalized the dangerousness, both the incumbent and the challenger would be correct. If this issue were the deciding factor in the election, which candidate wins would come down to the weighting of which outcome was more relevant to voters: aggregate danger or danger due specifically to homicide[4].
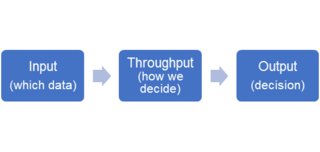
Although this example is fictitious, it shows how conclusions can vary based on numerous factors. We can portray this using a general input, throughput, output model (Figure 1), where input concerns which data are used, throughput concerns how we weight that data and the specific strategies we use to decide, and the output is the decision itself. Altering the inputs may alter the resulting decision (e.g., considering only X may result in one decision choice, but considering X and Y may result in a different decision choice), as might altering the throughputs (using decision strategy A may result in one decision choice, but using decision strategy B may result in a different decision choice).
This is a primary reason why Kolsky (2012) argued that all data is contextual. Data often provides little insight if we fail to understand the ways in which both the inputs and the throughputs affected the conclusions drawn from that data.
So, the next time someone argues “the evidence is clear that X” (or something comparable), ask yourself whether the context (i.e., input and throughput) used to adduce X is reasonable or whether the context was specifically selected in order to adduce X (as opposed to Y or Z). That could alter your decision of whether to accept or reject X.
So, when deciding which population center is more dangerous or which mayor to vote for, remember that the data you use to draw these conclusions are based on some specific context, a context that might be more subjective that you realize.
References
Footnotes
[1] Every inference I draw from Fox’s article is limited to that period (so it’s possible those inferences would not be the same, given we are in 2022.
[2] Higher risk from these two causes of death is a trend that has existed for over 20 years, so it’s not a recent development
[3] While urban life may not be more dangerous in a broader sense than other population centers, that is certainly not the only criterion we use to decide where to live.
[4] Assuming, of course, that voters were not overly swayed by their own politically-driven ideological biases, as we see in many elections (Lachat, 2008; Martin, 2022). If they are, then no matter which risk is more relevant to voters, they may vote based on their bias.