Education
The Right Level of Struggle for Learning
Why getting it right 85 percent of the time can be just right.
Posted November 5, 2019
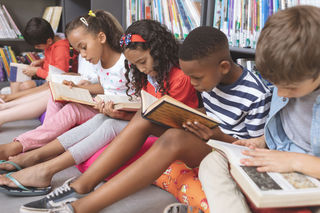
When children are learning to read, teachers often suggest a rule for picking an appropriate book. Count the words on the page that you don’t know. Five words? The book is just right. More? It’s too hard. Fewer? It’s too easy, and you’re not challenging yourself.
A team of scientists from the University of Arizona, Brown, UCLA, and Princeton has just applied machine-learning to that kind of thinking. They wanted to assess exactly how difficult training should be in order to improve learning. They found that there is an 85 percent rule for optimal learning. Or put another way, making errors about 15 percent of the time represents “a sweet spot” where you are going to be learning the most.
Conditions apply, of course. The specific percentages, which came from simple tasks run on a computer, matter less than the principal that such a sweet spot exists, says psychologist and cognitive scientist Robert Wilson of the University of Arizona, the lead author of the new paper, published Nov. 5 in Nature Communications.
“Training at perfection is not going to be a good thing,” Wilson says. The results also apply to a particular kind of learning, the kind where we learn from repetition over time. I talked with Wilson about this kind of learning and the study’s findings.
Optimal learning is an old idea, isn’t it?
There’s a long literature on learning in humans, in animals, and more recently in machines. This goes back to the '30s and [a Soviet psychologist named] Lev Vygotsky in education who coined this term, the “zone of proximal development” for learning. His observation was that kids tend to learn best when they are given a task that’s just at the edge of their competence. His idea was that’s how we should be teaching people, giving them tasks where they have to struggle a little.
Tell me about your study.
What we’ve done here is create a mathematical theory capturing some of these points. To do that, we’ve made some simplifications. We’ve focused on binary classification tasks, and [we used] a particular kind of algorithm called a gradient descent learning algorithm. It’s related to trial and error learning.
Learning means changing the parameters inside your brain or the parameters inside your model to reduce your error rate, on average. With more and more training, [a human or a computer model] learn to do better. One example might be a dermatologist learning to recognize whether a mole is cancerous or not. You can be formally taught what the characteristics of a cancerous mole are, but you get better at it by having a lot of experience in making those kinds of judgments over and over and getting feedback on whether it’s correct or not.
What does that mean for kids and learning?
Math is like this. You can teach someone the rules of it, but then to get better at it, you have to practice and practice. Playing a sport is the same thing. You can teach someone how to hold a baseball bat, but you don’t get good until you’ve practiced it a lot of times. It’s that kind of learning where it’s slow, and you’re gradually improving your performance on the task.
You did a machine-learning version of this?
We took algorithms where we know exactly what the machine is doing and how it’s learning. Then we constrain the problem to be very, very simple.
We simulated it on three different tasks. One is a very artificial example. We arbitrarily defined two different patterns of 1s and 0s as category 1 and category 2, and we trained the network to make that classification. Then we used a data set of about 60,000 images of handwritten numbers from zero to nine. We [asked the computer to distinguish] an even number or an odd number, or a high number, greater than 5, or a low number, less than 5.
We ranked the images on how difficult they were. That allowed us to monitor the training accuracy of the algorithm as it’s learning. As its accuracy starts to go up, we give it harder examples. If the accuracy goes down, then we start to give it easier examples again. We tested a range of different accuracies, and we found that the fastest training was at 85 percent.
The last task was a perceptual learning task that’s famous in psychology—the dot motion task. You see a bunch of randomly moving dots on the screen. The trick is that some are moving left or right, but most are moving randomly. You’ve got to decide, is it [on average] moving left or right? It’s a task you get better at with experience. We trained a computer model at a bunch of different training accuracies. Again, 85 percent accuracy was the most efficient learning.
How can knowing this number, 85 percent, improve learning in the real world?
Having a number that’s optimal can improve the rate of learning. You can train your algorithm faster to a certain level of accuracy. That’s always good from that practical perspective. The hope is this applies to human learning and that we can find this sweet spot.
The more general idea that there is a provable sweet spot is helpful to frame the other findings in this historical literature. But it’s also pointing toward a theory that maybe we can extend this to different kinds of learning and different kinds of learning problems. There’s some work going on where people have started trying to design things like math curriculum, where you do it on a computer, and it adapts the difficulty of the problem based on the needs of the student.
You also connected your findings to the concept of flow. Can you explain?
We speculate that 85 percent is not only optimal for learning. Maybe that’s the place where you’re more likely to get into this flow state, where your skill level matches your challenge. What was striking to me was that the original model of flow has three different states—anxiety, flow, and boredom. They really map on to [our] model in a one-to-one way.
The flow state is where your skill matches your challenge and that’s where we predict the fastest learning. Boredom is where you’re not learning, and your accuracy is at 100 percent. And anxiety is where you’re not learning, and your accuracy is at 50 percent or chance. This is pure speculation, but that’s something we’re excited to think about going forward.
What do these findings tell us about how the brain learns?
[Up close], the learning process looks largely the same regardless of the training accuracy. You’d learn faster at 85 percent accuracy, but it would be hard to know you're learning faster just looking at individual synapses.
However, the speculation is that people have some awareness of their own learning progress, either implicitly or explicitly. Flow may involve a distinct brain state: When I'm learning optimally, I'm in this brain state associated with flow and that's pleasurable, so I seek out more tasks at this intermediate difficulty level and learn more.
Copyright: Lydia Denworth, 2019