Artificial Intelligence
The Neuroscience Origins of AI Computer Vision
How cats from over 60 years ago led to AI tech that may drive your car.
Posted March 2, 2021
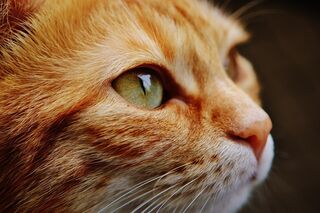
The artificial intelligence (AI) renaissance is in full steam and gathering speed. One of the key levers of momentum is computer vision, an interdisciplinary science that spans artificial intelligence, physics, neuroscience, biology, statistics, learning theory, engineering, statistics, robotics, and mathematics. Biological vision has inspired artificial intelligence machine learning vision.
The computer vision global market size is projected to reach USD 19.1 billion by 2027, growing at a CAGR of 7.6 percent during 2020-2027 according to Grand View Research’s September 2020 report. Computer vision is the enabling technology fueled by AI behind autonomous vehicles, sports analytics, radiology, medical diagnostics, agricultural yield predictions, manufacturing maintenance, retail loss prevention, security surveillance, fraud prevention, and more uses.
Yet the history of computer vision is relatively recent and rooted in the decidedly biological realm. Back in 1959, neurophysiologists David Hubel and Torsten Wiesel published their landmark paper titled “Receptive fields of single neurons in the cat's striate cortex” in the Journal of Physiology. Neurophysiology is the branch of neuroscience and physiology that is the scientific study of the brain and peripheral nervous system.
By studying vision in cats, the Hubel and Wiesel discovered that neurons in the visual cortex have a distinct arrangement. The arrangement of the receptive fields determines the orientation, form, and size of the stimuli, and may play a role in the perception of movement.
In 1968 the same research duo published a study on the receptive fields and functional architectures of the striate cortex of monkeys that showed that most cells can be categorized as simple or complex. Simple cells in the primary visual cortex mostly respond to oriented edges and bars of particular orientations. Complex cells have a receptive field that integrates and sums the input of simple cells, a foundational concept for convolutional neural network models for computer vision. Hubel and Wiesel were among the recipients that shared the Nobel Prize in Physiology or Medicine in 1981.
Influenced by Hubel and Wiesel’s work, in 1980 Japanese computer scientist Kunihiko Fukushima published in Biological Cybernetics the concept of a self-organizing neural network model as a mechanism of visual pattern recognition called the Neocognitron. Using mathematics, Fukushima recreated the concept of the simple and complex cells to create a computational model for computer vision. When the Neocognitron network completes self-organization, its architecture resembles Hubel and Wiesel’s hierarchical model of the visual nervous system.
The Neocognitron recognizes stimulus patterns based on geometrical similarity, or Gestalt, of their shapes. In the German language, gestalt roughly translates to a unified whole. In the 1920s, German psychologists Max Wertheimer, Kurt Koffka, and Wolfgang Kohler founded Gestalt psychology to explain perception with the fundamental concept that the whole is greater than the sum of the parts.
In 1998, researchers Yan LeCun, Leon Bottou, Yoshua Bengio, and Patrick Haffner made AI history with their breakthrough study published in the Proceedings of the IEEE called “Gradient-Based Learning Applied to Document Recognition” that shows the effectiveness of using Convolutional Neural Networks (CNNs) for handwriting character recognition. The researchers demonstrated that using gradient-based learning to convolutional neural networks enables the learning of relevant features from training data.
"Convolutional Neural Networks have been shown to eliminate the need for hand-crafted feature extractors,” wrote LeCun, Bengio, and et al. in their study. “Graph Transformer Networks have been shown to reduce the need for hand-crafted heuristics, manual labeling, and manual parameter tuning in document recognition systems. As training data becomes plentiful, as computers get faster, as our understanding of learning algorithms improves, recognition systems will rely more and more of learning, and their performance will improve.”
In 2012, AI pioneers Alex Krizhevsky, Ilya Sutskever, and Geoffrey Hinton presented their groundbreaking paper, “ImageNet Classification with Deep Convolutional Neural Networks” at the NeurIPS conference that showed record-breaking computer classification of 1.2 million high-resolution images.
By 2027, the global market size for deep neural networks alone is projected to reach USD 5.98 billion, growing at a CAGR of 21.4 percent during 2020-2027, according to Emergen Research’s September 2020 report. The prominent players in deep neural networks include Google, IBM, Microsoft, Qualcomm, Intel, Oracle, Clarifai, Neurala, NeuralWare, Starmind, and Ward Systems, per the same report.
What started out as a fundamental discovery over 60 years ago in a research study with cats has formed the foundational concept for modern AI-enabled computer vision, a multi-billion-dollar industry. That’s how a pair of Nobel Prize-winning neurophysiologists have created the scientific thread that led to enabling technologies that may eventually drive your car for you in the future.
Copyright © 2021 Cami Rosso All rights reserved.