There is an old joke about a scientist and a flea. The scientist put a flea on the table, then smacked his hand heavily on the table and the flea jumped. The scientist next tore off two of the flea’s legs and smacked again, and again the flea jumped. The scientist tore off two more legs, repeated the procedure, and again the flea jumped.
The scientist tore off the last two legs, smacked his hand on the table, and — no jumping. He tried again, smacked his hand heavily on the table, but the flea still didn’t jump.
The scientist wrote down his observation: “When a flea loses all its legs, it becomes deaf.”
Similarly, if you take experts and put them in a situation where they have to perform an unfamiliar task (two legs off), and remove any meaningful context (two more legs off), and apply an inappropriate evaluation criterion (last two legs off), it’s a mistake to conclude that experts are stupid.
I was reminded of this joke when I read some accounts of how advanced Artificial Intelligence systems were outperforming experts. For example, in healthcare, a diagnostician treating a patient might look at an x-ray for signs of pneumonia, but AI systems can detect pneumonia in x-rays more accurately. Or the physician might study the results of a battery of blood tests, but AI systems can detect problems from Electronic Health Records more accurately than physicians.
What’s missing from this picture is that the physician also has a chance to meet patients and observe them — how they are moving, especially compared to the last office visit. How they are breathing, and so forth. The AI systems don’t have a way to take these observations into account and so the comparative studies screen out any observation and require the physicians to base their judgments entirely on the objective records. That’s two legs off. The physicians aren’t allowed to consider any personal history with the patients — another two legs off. The physicians can’t consult with family members — a final two legs off. And so the researchers conclude that the physicians aren’t very skillful — not as accurate as the AI.
I think what we need is a way for the AI developers to enhance the physicians’ judgments, not replace them. Here is an example, a study by Wang et al. (2016). The pathologists’ error rate was 3.5 percent, whereas the AI model error rate was only 2.9 percent. A clear win for the AI model it would seem. However, the combined error rate, adding the pathologist to the AI, was 0.5%.
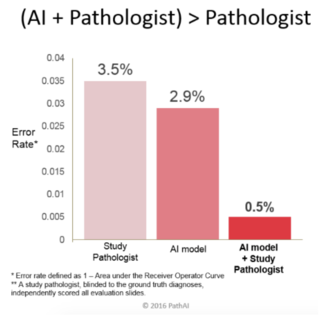
Another study (Rosenberg et al., 2018) describes how an AI-powered mechanism used “swarm intelligence” among a group of expert radiologists reviewing chest x-rays for the presence of pneumonia. The swarm beat the standard radiologist performance by 33 percent but it also beat Stanford’s state-of-the-art deep learning system by 22 percent.
Siddiqui (2018) has described another example of human/AI partnering. Experienced physicians can identify the one in a thousand very sick child about three-quarters of the time. In order to increase accuracy of detection and reduce the number of children being missed, some hospitals are now using quantitative algorithms from their electronic health records to choose which fevers were dangerous. The algorithms are relying entirely on the data and are more accurate than the physicians, catching the serious infections nine times out of ten. However, the algorithms had ten times the false alarms. One hospital in Philadelphia hospital took the computer-based list of worrisome fevers as a starting point, but then had their best doctors and nurses to look over the children before declaring the infection was deadly and bringing them into the hospital for intravenous medications. Their teams weeded out the algorithm’s false alarms with high accuracy. In addition, the physicians and nurses found cases the computer missed, bringing their detection rate of deadly infections from 86.2 percent by the algorithm alone, to 99.4 percent by the algorithm in combination with human perception.
So it is easy to make experts stupid. But it is more exciting and fulfilling to put their abilities to work.
I thank Lorenzo Barberis Canonico for bringing these studies to my attention.
References
Rosenberg, L., Willcox, G., Halabi, S., Lungren, M., Baltaxe, D. & Lyons, M. (2018). Artificial Swarm Intelligence employed to Amplify Diagnostic Accuracy in Radiology. . IEMCON 2018 – 9th Annual Information Technology, Electronics, and Mobile Communication Conference
Siddiqui, G. (2018). Why doctors reject tools that make their job easier. Scientific American, Observations newsletter, October 15, 2018.
Wang, D., Khosla, A., Gargeya, R., Irshad, H., Beck, A.H., (2016). Deep learning for identifying metastatic breast cancer. Unpublished paper.