Psychiatry
Can Artificial Intelligence Improve Psychiatric Diagnosis?
New research on adult psychiatric illness uses advances in machine-learning.
Posted February 13, 2018
I believe this artificial intelligence is going to be our partner. If we misuse it, it will be a risk. If we use it right, it can be our partner.
—Masayoshi Son
Sadly, for many people current psychiatric and psychological approaches do not provide sufficient clarity or relief. Unlike many traditional "physical" illnesses, which are often better characterized (though many still defy understanding), psychiatric illness presents challenges to medicine because of the massive complexity of the brain and mind, making it historically difficulty to develop tools for assessment and treatment. Until relatively recently, diagnostic categories were based on clinical observation and statistical analysis of symptoms and treatment responses, making for an imperfect science. While this situation still holds true to a very significant extent, at the dawn of the 21st century we began to see the development of more powerful tools as a result of advances in assessment and computational analysis, giving hope for future improvement. By better understanding brain function in wellness and illness, and making biological connections to psychology and mind, the body-mind, physical-mental split is slowing being bridged.
The state of the art.
One of the limitations of current psychiatric diagnosis is that many conditions overlap. Anxiety, mood disturbances, fear, difficulty with concentration and memory, energy level changes, and a variety of other symptoms are shared across many diagnoses. At least 50 percent of patients receive more than one psychiatric diagnosis, which sometimes is the result of diagnostic murkiness and sometimes the result of actual co-occurring conditions.
People visiting more than one provider may be diagnosed differently, leading to confusion, straining trust, and complicating recovery planning. Developing more accurate models of mental health is imperative, given that anxiety and depression cause the greatest lost productivity and burden on function worldwide (e.g. World Health Organization), and current treatments generally are very effective for only 30 percent of patients. There is a clear need for more accurate diagnostic approaches, for developing valid biological tests ("biomarkers") and for linking diagnosis with more effective treatments and treatment plans. While the current diagnostic nomenclature represents the best effort to date, new methods are becoming available for better understanding psychological health.
Approaching psychiatric diagnosis by using mathematical tools to search for consistent patterns inherent in clinical data promises advantages over conventional diagnostic approaches, which are subject to error from human bias (in spite of statistical analysis) and the risk of holding onto familiar categories in favor of more accurate approaches, People tend to resist change, and while newer is not always better, being open to thoughtfully novel approaches moves healthcare forward. Machine-learning is a powerful tool for looking at massive data sets and discovering useful patterns in data which other techniques miss. By using AI-type approaches, researchers can leverage computation power to see consistencies in how symptoms cut across received diagnostic categories to develop “transdiagnostic” perspectives. While this has to an extent been done with adolescents, machine-learning has not be applied to adult psychopathology.
Toward a transdiagnostic approach to adult psychiatry.
In order to begin to identify transdiagnostic features of different disorders in adults, Grisanzio and colleagues (2018) worked with 497 adults of diverse demographic backgrounds, including 248 with major depressive disorder, PTSD and panic disorder, and 249 people without psychiatric diagnoses. They chose these three diagnoses to work with because they are common, and participants often had received additional diagnoses including ADHD, generalized anxiety disorder, obsessive-compulsive disorder, dysthymia and seasonal affective disorder. They excluded participants with substance use disorder, brain injury, and other conditions which would interfere with testing procedures.
They assessed participants using accepted diagnostic tools including the Hamilton Depression Rating Scale, the Structured Clinical Interview for DSM-IV, and others; self-report scales for psychiatric symptoms measuring moods, anxiety, stress, self-esteem, hopelessness, and myriad other symptoms using the Depression, Anxiety and Stress Scale; a neurocognitive battery (IntegNeuro) to assess cognitive function; EEG (electroencephalogram) to assess basic brain activity; and daily functional capacity using the Brief Risk-Resilience Index for Screening.
Data were analyzed first using “principal component analysis” to identify trends in the main clinical measures, followed by an “unsupervised” analysis using a machine-learning approach which does not require human input, but rather independently identifies significant clusters present within the data. Finally, in addition to testing the main group of 497 participants, they repeated the same measures on a completely different group of 381 adults, to provide an “independent validation sample” to confirm the validity of the results. Their findings held true for both the primary test subjects as well as the independent validation group, suggesting robust applicability.
In the basic analysis (“principal component analysis” they found that 3 factors accounted for the majority (71.2 percent) of the clinical data: anhedonia, anxious arousal, and tension. These 3 factors represent clinical symptoms across the three main diagnostic categories and co-occurring conditions. The unsupervised machine-learning analysis yielded 6 independent clusters: normative mood (the healthy control group), tension, anxious arousal, general anxiety, anhedonia, and melancholia. Here’s what that machine-learning process looks like graphically:
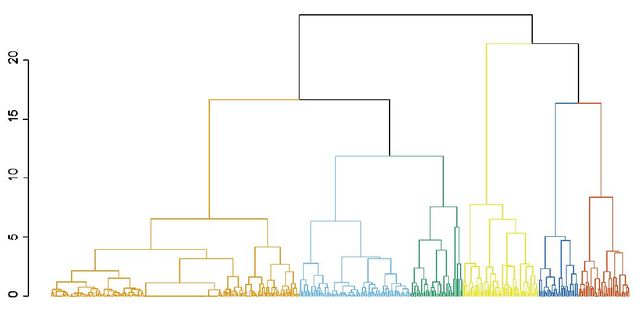
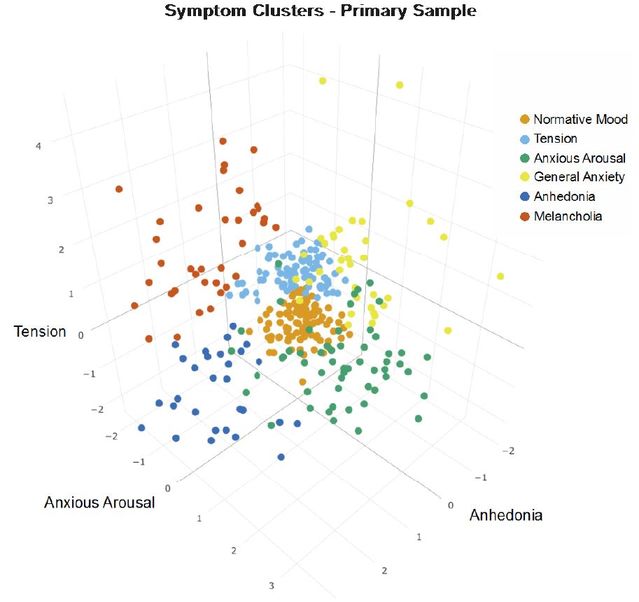
Each of these clusters represents statistically different groups of symptoms, with unique fingerprint combining different clinical presentation, neurocognitive markers, EEG activity and functional status. In addition to having different clinical characteristics, the 6 clusters differed notably:
- Anxious arousal: Poor daily functioning, greatest neurocognitive difficulty, especially impaired cognitive control.
- General anxiety: Higher EEG responses in the beta-bandwidth (usually associated with more higher brain activity) in parietal cortex, and intact daily function.
- Melancholia: Poorest daily function, especially socially.
- Anhedonia: Higher beta activity in the frontal cortex.
- Tension: Average performance across the boards in spite of high levels of stress.
How do these 6 clusters transdiagnostically relate to the conventional psychiatric diagnoses? This figure shows where the clusters overlap with the diagnoses we currently use:
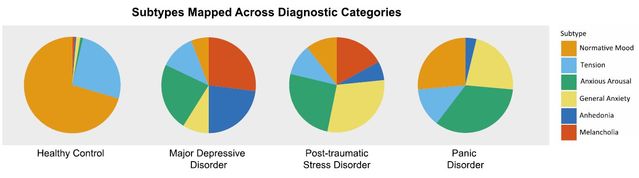
Does psychiatric care have a brighter future?
While this research is useful for understanding underlying symptoms for common psychiatric conditions from a data-driven perspective, the implications of this early transdiagnostic study of adults open the door to further refinement in clinical application. Current diagnostic categories are blurry, overlapping with one another, and may not fully capture the best picture of what is going on for a given individual. With ongoing research to further confirm and expand upon the current findings, we may have a more accurate way of assessing mental health, which should be better for treatment planning.
Future studies will include additional diagnostic categories, and use assessment tools such as functional neuroimaging to gain more detailed knowledge, and tools for more accurate diagnosis. Combined with a greater understanding of mechanisms of disease, and research on assessment and treatment, we are beginning to see better tools, such as pharmacogenomic testing, TMS, novel drugs, and third-generation psychotherapeutics, among others. Eventually, we hope to be able to develop a biologically-based system for giving relief to people suffering from psychiatric illnesses, in terms of assessment, treatment, and especially for future generations, prevention.
References
Grisanzio KA, Goldstein-Pierkarski AN, Wang MY, Ahmed APR, Samara Z & Williams LM. Transdiagnostic Symptom Clusters and Associations
With Brain, Behavior, and Daily Function in Mood, Anxiety,
and Trauma Disorders.JAMA Psychiatry. 2018;75(2):201-209. doi:10.1001/jamapsychiatry.2017.3951
Published online December 3, 2017. Corrected on February 7, 2018.