Artificial Intelligence
Brain-Inspired Nanowire Network May Revolutionize AI
New AI neuromorphic computing system learns handwriting with high accuracy.
Posted November 16, 2023 Reviewed by Gary Drevitch
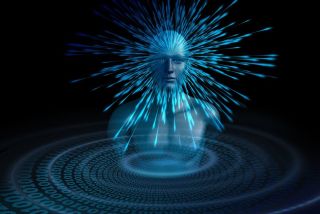
Artificial intelligence (AI) machine learning algorithms consist of neural networks that are inspired by the biological brain. However, today’s standard computing hardware architecture is not, hence running AI on conventional hardware is currently energy inefficient. Exciting new research may help bridge this gap. A study by researchers at the California NanoSystems Institute (CNSI) at UCLA along with their collaborators at the University of Sydney show a brain-inspired nanowire network AI computing system that can identify handwritten numbers with high accuracy.
“In this study, the relatively high classification accuracy achieved with online learning (93.4%) can be largely attributed to the iterative algorithm, which is based on recursive least squares (RLS),” wrote first author Ruomin Zhu, a doctoral student at the University of Sydney, along with co-authors James Gimzewski, UCLA distinguished professor of chemistry and CNSI member, Adam Stieg, CNSI associate director and research scientist at UCLA, Zdenka Kuncic, professor of physics at the University of Sydney, Alon Loeffler, of the University of Sydney, Joseph Lizier, associate professor at the University of Sydney, and Sam Lilak, an experimental physicist from UCLA.
In AI machine learning, recursive least squares (RLS) are a type of adaptive filter algorithm known for faster convergence and higher performance. When compared to least mean squares (LMS), recursive least squares algorithms are more complex and therefore more costly to run. RLS algorithms use a recursive approach for adaptation in which the objective is to minimize the total weighted squared error between the target signal and output.
For this research study, the team used a class of neuromorphic devices consisting of memristive nanowire networks (NWN), metal-based nanowires networked in a design inspired by the biological brain.
Nanowires are very thin solid wires that may be made from a variety of materials that typically have diameters up to 100 nanometers. Nanowires are highly flexible and strong. For this study, the researcher team created a brain-inspired hardware architecture with nanowires made of silver and selenium (Ag2Se) with room-temperature transport properties such as glass-like thermal conductivity and good electrical conductivity.
The network of nanowires are laid on a multi-electrode array (MEA) consisting of 16 electrodes on a 4-by-4 grid where input and output are produced with pulses of electricity. When electrical input signals are made, the nanowire networks act like the biological brain with collective dynamics such as avalanche criticality, phase transitions, and switch synchronization.
The nanowire network was trained on a handwritten number database from the National Institute of Standards and Technology (NIST). Through electrical pulses of one-thousand of a second duration, images were transmitted to the nanonetwork pixel by pixel. Dark or light pixels were achieved by adjusting the voltage of the electrical pulses.
“In conclusion, we have demonstrated how neuromorphic nanowire network devices can be used to perform tasks in an online manner, learning from the rich spatiotemporal dynamics generated by the physical neural-like network,” the scientists reported. “This is fundamentally different from data-driven statistical machine learning using artificial neural network algorithms.”
Copyright © 2023 Cami Rosso All rights reserved.