Artificial Intelligence
Are AI Models Like ChatGPT Rational?
A new study applies human cognitive psychology to AI with unexpected results.
Posted June 11, 2024 Reviewed by Ray Parker
Key points
- A new study uses cognitive psychology tests to examine the rationality of large language models (LLMs).
- The study found that LLMs can be irrational, but their irrationality differs from human irrationality.
- The irrationality of LLMs has safety implications, especially in fields like medicine and diplomacy.
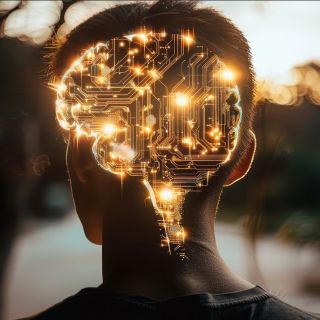
The release of ChatGPT by OpenAI to the general public in November 2022 has brought the capabilities of large language models (LLMs), such as those that power the popular artificial intelligence (AI) chatbot, into the limelight. Can humans trust the output from these AI neural networks? Are AI large language models rational? A new study by researchers at the University College London (UCL) uses cognitive psychology to examine the rationality of AI large language models with thought-provoking results.
“We evaluate the rational reasoning of seven LLMs using a series of tasks from the cognitive psychology literature,” wrote Mirco Musolesi, Ph.D., professor of computer science and corresponding author Olivia Macmillan-Scott at the University College London.
The cognitive psychology tests used for this study were mostly developed from a series of tasks designed to spot human heuristics and biases by two pioneers in the field of psychology and behavioral economics—Daniel Kahneman (1934-2024), the late professor emeritus of psychology and public affairs at Princeton University and Amos Tversky (1937-1996), the late mathematical psychologist and professor at Stanford University.
Kahneman was known for his expertise in the psychology of decision-making and judgment. He was one of the recipients of The Sveriges Riksbank Prize in Economic Sciences in Memory of Alfred Nobel 2002 "for having integrated insights from psychological research into economic science, especially concerning human judgment and decision-making under uncertainty,” according to The Nobel Foundation. Kahneman wrote the New York Times bestseller Thinking, Fast and Slow, which was released in 2011.
Kahneman and Tversky's paths crossed in the late 1960s, and in the following decades, they published research on cognitive psychology, which was used for this new study. The research covered subjective probability, judgment under uncertainty, heuristics, biases, extensional versus intuitive reasoning, and the psychology of preferences.
Out of the dozen cognitive tasks, a majority, nine out of 12, were developed by Kahneman and Tversky, and the remaining three were by Peter C. Wason (1924-2003), a UCL cognitive psychologist and pioneer in the psychology of reasoning, David M. Eddy (1941-), a physician and mathematician, and Daniel Friedman, a professor of economics.
“Humans predominantly respond to these tasks in one of two ways: they either answer correctly, or they give the answer that displays the cognitive bias,” wrote the UCL researchers.
Specifically, the tasks used for this study to identify cognitive biases include the Wason task (confirmation bias), AIDS task (inverse/conditional probability fallacy), hospital problem (insensitivity to sample size), Monty Hall problem (gambler’s fallacy, endowment effect), Linda problem (conjunction fallacy), birth sequence problem (representativeness effect), high school problem (representativeness effect), and marbles task (the misconception of chance). Each model was prompted 10 times by the researchers in order to determine the consistency of the performance of the LLMs, and each LLM model response was categorized for accuracy (correct answers or not) and whether the answer was human-like or not.
The UCL researchers evaluated large language models by OpenAI (GPT-4, GPT 3.5), Google (Bard), Anthropic (Claude 2), and Meta (Llama 2 model 7B, Llama 2 model 13B, Llama 2 model 70B). The team used the OpenAI application interface to prompt GPT and the online chatbot for the other LLMs.
According to the scientists, OpenAI’s GPT-4 outperformed all the other models by providing the correct response and reasoning in over 69% of cases, with Anthropic’s Claude 2 model ranking second best on the same criteria in 55% of cases. On the other hand, Meta's Llama 2 model 7 b performed the worst and gave the most incorrect responses in over 77% of cases.
“We find that, like humans, LLMs display irrationality in these tasks,” the researchers shared. “However, the way this irrationality is displayed does not reflect that shown by humans.”
When applying the same set of tasks to the select LLMs in this study, the researchers discovered that the LLMs are “highly inconsistent”—the same model can give both correct and incorrect answers and human-like and non-human-like answers in separate runs. An interesting discovery is that most of the incorrect responses are incorrect in ways that are not human-like biases.
“It is interesting to note that across all language models, incorrect responses were generally not human-like, meaning they were not incorrect due to displaying a cognitive bias,” the UCL scientists pointed out. “Instead, these responses generally displayed illogical reasoning, and even on occasion provided correct reasoning but then gave an incorrect final answer.”
In short, the UCL researchers have demonstrated in this study that the LLMs have an irrationality that is different from human irrationality. The researchers point out that the irrationality of AI large language models has safety implications for certain fields such as medicine and diplomacy.
The scientists conclude that their methodology can go beyond assessing rational reasoning and cognitive biases; it has the potential to be used more broadly to evaluate other cognitive capabilities of artificial intelligence large language models in the future.
Copyright © 2024 Cami Rosso. All rights reserved.