Brain Computer Interface
AI Predicts Behavior From Brain Activity
AI deep learning accelerates brain-computer interface (BCI) neurotech accuracy.
Posted April 4, 2024 Reviewed by Ray Parker
Key points
- Brain-computer interfaces use brain signals to control external devices.
- Deep learning is a type of machine learning where algorithms learn from data without explicit instructions.
- A new study shows that AI helps predict behavior with brain scans.
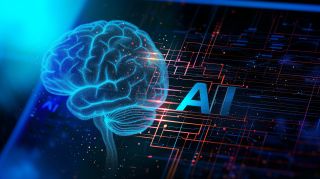
Neuroscience, behavioral science, and medical research are accelerating with the help of the predictive power of artificial intelligence (AI) machine learning. A new peer-reviewed study published in PLoS Computational Biology demonstrates an AI deep learning model that can predict behavior at nearly real-time speed with 95% accuracy.
“Deep learning is a powerful tool for accurately decoding movement, speech, and vision from neural signals from the brain and for neuroengineering such as brain-computer interface (BCI) technology that utilizes the correspondence relationship between neural signals and their intentional behavioral expressions,” reported corresponding author Toru Takumi at the Kobe University School of Medicine, along with researchers Takehiro Ajioka, Nobuhiro Nakai, and Okito Yamashita.
Brain-computer interfaces enable those with impaired motor or speech and other disabilities to operate and control external devices such as computers, robotic limbs, as well as communicate. For those suffering from neurological disorders, locked-in syndrome, motor impairment, and paralysis, brain-computer interfaces offer hope of improving the quality of daily life.
Deep learning is a subset of machine learning. In machine learning, algorithms “learn” from massive amounts of training data rather than rely on explicit hard coding of instructions. A deep neural network consists of an input layer, an output layer, and many layers of artificial neural networks in between. Deep learning algorithms are responsible for the ongoing AI renaissance.
The Kobe University School of Medicine researchers’ AI model design consists of a convolutional neural network (CNN) for image data analysis and a recurrent neural network (RNN) for processing sequential, time-variable data. The researchers call this approach an “end-to-end” deep learning.
Convolutional neural networks are deep learning neural networks often used for image classification for computer vision tasks and natural language processing. A convolutional neural network is a feed-forward network that does not require any preprocessing and excels at identifying patterns in raw image data. Like the human visual cortex, a convolutional neural network uses a series of processing layers to spot features with greater complexity progressively. With more convolutional layers, more complex features can be identified.
A recurrent neural network is a bi-directional deep learning algorithm that uses time series or sequential data to perform tasks such as image captioning, speech recognition, translation, and natural language processing. In recurrent neural networks, the output is dependent on prior inputs within the sequence. Instead of traditional backpropagation, recurrent neural networks use backpropagation through time (BPTT) to sum errors at each step.
In addition to real-time speed and high accuracy, what distinguishes the Kobe University School of Medicine’s AI deep learning model is that it does not require the time-consuming data preprocessing task of selecting areas of interest. The neuroscientists evaluated their AI model for behavioral classification, specifically to determine whether laboratory mice were resting or moving on a treadmill using whole-cortex brain images without predefining brain areas of interest. The researchers ran the AI model on five mice and discovered that it was able to generalize and screen out individual attributes.
“Our findings demonstrate possibilities for neural decoding of voluntary behaviors with the whole-body motion from the cortex-wide images and advantages for identifying essential features of the decoders,” reported the Kobe University School of Medicine research team.
To understand what areas the AI model identified as areas of interest, the researchers used a methodical process of elimination. The team methodically eliminated portions of the imaging data and evaluated the AI deep learning model’s performance. In this manner, the neuroscientists were able to isolate and determine which image data contributed the most to the AI model’s prediction accuracy. This helps to illuminate the proverbial “black box” of AI deep learning by showing what data impacted the AI model’s performance the most.
“To make deep learning decoding interpretable, we tried to quantify the critical areas of images that contributed to the behavioral classification in the CNN-RNN decoder,” wrote the researchers.
The Kobe University School of Medicine neuroscientists have successfully provided proof-of-concept of combining two AI deep learning algorithms to quickly and accurately decode neural activity that may one day help advance human brain-computer interface technology.
Copyright © 2024 Cami Rosso All rights reserved.