Artificial Intelligence
Scientists Create Human-like AI Computer Vision
New AI deep learning algorithm “sees the big picture” from only a few glances.
Posted May 29, 2019
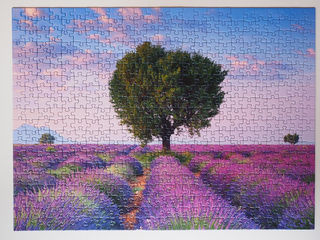
Two weeks ago computer scientists at the University of Texas at Austin (UT Austin) published a study in Science Robotics that demonstrates an artificial intelligence (AI) system with the human-like ability to “see the big picture” from only a few glances.
Recent progress in image pattern recognition, and computer vision, are mainly due to the rise of AI deep learning. Computer vision is typically achieved through training deep learning algorithms for a specific purpose. But this method produces a custom system that is inflexible and slow to train. UT Austin professor Kristen Grauman led a study with team members Santhosh Ramakrishnan, Ph.D. candidate, and Dinesh Jayaraman, Ph.D. candidate (now at the University of California, Berkeley), with the goal of creating AI that is faster and more versatile than standard computer vision systems.
To achieve these objectives, the researchers trained their algorithm at UT Austin’s Texas Advanced Computing Center and Department of Computer Science using AI reinforcement learning. Similar to the behavioral psychology concept of reinforcement, in which consequences are used to modify behavior, in AI reinforcement learning, the algorithm learns by interacting with its environment and is rewarded for performing correctly or penalized for performing incorrectly. For example, in an autonomous vehicle, an algorithm receives a reward state for stopping at a red traffic light, or a penalty state for going the wrong direction on a one-way road.
In this study, the scientists rewarded the algorithm for reducing uncertainty about the unobserved parts of an environment. The algorithm was trained to select a short sequence of glances, then infer the appearance of the entire environment. Given that this approach produces scanty rewards, the team created another algorithm, called a “sidekick policy learning agent," to speed up the training.
“We use the name 'sidekick' to signify how a sidekick to a hero (e.g., in a comic or movie) provides alternate points of view, knowledge, and skills that the hero does not have,” wrote researchers Grauman and Ramakrishnan in their paper, “Sidekick Policy Learning for Active Visual Exploration,” which was presented in 2018 at the European Conference on Computer Vision (ECCV). Unlike the main algorithm, a sidekick “complements the hero (agent), yet cannot solve the main task at hand.”
For example, if a shopper was searching for puffy vests at a new department store, and found a display of pots and pans, it is reasonable to assume that there are more housewares adjacent, and therefore choose to glance in the other direction as a next step in the search rather than nearby. A personal shopper would act like a sidekick and provide assistance to the shopper to speed the search.
This new algorithm is general use—suitable for a broad range of purposes. The ability to size up an environment quickly based on a few glances is particularly useful in search-and-rescue situations where time is limited. The researchers plan to develop their system to work in mobile robots in the future.
Copyright © 2019 Cami Rosso All rights reserved.
References
Ramakrishnan, Santhosh K., Jayaraman, Dinesh, Grauman, Kristen. “Emergence of exploratory look-around behaviors through active observation completion.” Science Robotics. 2019.
UT News (2019, May 15). New AI Sees Like a Human, Filling in the Blanks [Press Release]. Retrieved https://news.utexas.edu/2019/05/15/new-ai-sees-like-a-human-filling-in-…