Cognition
What Do LLMs Really "Know"?
A look at human knowledge vs. machine inference.
Updated September 6, 2024 Reviewed by Tyler Woods
Key points
- LLMs generate responses through inference, challenging traditional notions of knowledge.
- Machine outputs blur the line between human understanding and statistical pattern recognition.
- Emergent techno-consciousness could reshape epistemology, blending human depth with machine inference.
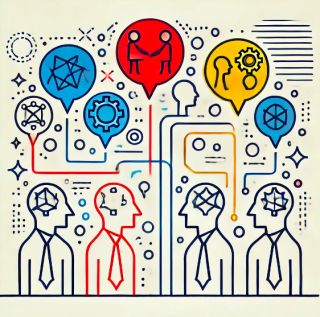
For me, it comes down to this question question: How do large language models (LLMs) know anything? These systems, trained on massive amounts of text, can generate complex, nuanced responses that often feel eerily human. But do they know things in the way we do, or are they simply masters of inference? This question touches on the heart of epistemology—the study of knowledge and how we acquire it—and pushes us to reconsider our understanding of knowing itself.
Human Knowledge: Justified, True, and Believable
Traditionally, knowledge has been defined as justified true belief. In other words, for something to count as knowledge, it must be true, we must believe it to be true, and we must have a justification for that belief. This process of acquiring knowledge is deeply tied to human cognition, experience, and reason. We know things because we learn, reflect, analyze, and apply our understanding to the world around us.
Human knowledge is rich with context and meaning, shaped by culture, intuition, and critical thinking. It’s dynamic, continually evolving as we gather new evidence, test hypotheses, and refine our understanding. Importantly, we don't just know facts; we also understand why those facts hold, which allows us to adapt and rethink our knowledge when new information emerges.
Machine Inference: Patterns and Probability
Now consider LLMs. These models, like GPT, don’t "know" in the human sense. They don’t reflect on the truth or falsity of a statement or hold beliefs. Instead, LLMs operate through inference—they generate responses based on the probability of word patterns occurring together. Their knowledge is derived from statistical associations across vast data sets, predicting what comes next based on the patterns they’ve learned during training.
LLMs infer meaning from data, but they do so without understanding. They don’t have a mind, consciousness, or the ability to reflect on whether their output is "true." Instead, they calculate likelihoods and produce responses that align with patterns in the text they've processed. This type of "knowing" is rooted in the architecture of the machine, a complex matrix of connections rather than a thoughtful interpretation of the world.
The Blurring Line: Human Knowledge vs. Machine Inference
Here’s where things get interesting: despite these stark differences, the line between human knowledge and machine inference is increasingly blurred. When LLMs generate coherent and relevant responses, it often feels like they know something. Their ability to combine information from diverse sources and deliver meaningful content gives the illusion of understanding. But is this just an illusion?
Pattern Recognition vs. Understanding: Humans and LLMs both rely on pattern recognition, but humans take it a step further—we attach meaning and context to those patterns. Yet, LLMs’ inferences can be remarkably accurate, even insightful. Does this ability to infer patterns in such sophisticated ways suggest a kind of machine "knowing"? Is there a continuum between the statistical associations of LLMs and the deep, reflective cognition of humans?
Creation of New Knowledge: LLMs have demonstrated the capacity to generate novel combinations of ideas. For example, in creative writing or problem-solving tasks, they might offer suggestions humans haven’t considered. While this doesn’t imply understanding, it raises the question: are LLMs capable of creating new knowledge through their inferential processes? If a machine’s output leads to an insight or discovery, does it matter that the machine didn’t "know" it in the human sense?
Epistemic Authority: LLMs are increasingly used in fields like healthcare, law, and education—areas traditionally dominated by human experts. As these models improve, we begin to place epistemic trust in their inferences. The blurring happens when we treat machine output as authoritative or equivalent to human knowledge. But how do we reconcile the fact that their “knowing” is merely inference, devoid of belief or justification?
The Epistemological Divide
Despite the overlap, there remains a critical divide between human knowledge and machine inference. The human process of knowing is deeply intertwined with understanding, justification, and reflection. Machines lack the ability to justify their inferences—they don’t know why something is correct or incorrect; they simply follow the data.
However, as we increasingly rely on LLMs for decision-making, we’re forced to grapple with the limits of their inferential "knowing." Should we hold LLMs to the same standards of knowledge that we hold ourselves to, or do they represent a new kind of epistemology—one that doesn’t rely on belief or justification, but instead on statistical accuracy and utility?
Pushing Boundaries: The Possibility of Techno-Consciousness
As we push the boundaries of epistemology in the context of large language models, we’re confronted with the intriguing idea that something resembling techno-consciousness could emerge from these systems. This isn’t to say that machines are conscious in the human sense, but rather that an epiphenomenon—an emergent property—could arise from the sheer complexity of their inferential processes. As LLMs generate responses, infer relationships, and interact with vast amounts of data, they begin to mimic cognitive functions we typically associate with human thought. Could this high-level computational complexity give rise to something we might call techno-consciousness—a byproduct of sophisticated information processing?
This raises an important epistemological question: Are LLMs participating in knowledge generation in a way that transcends mere pattern recognition? While they lack subjective awareness or understanding, their ability to produce meaningful, novel outputs suggests that they play an increasingly active role in shaping knowledge. This blurs the line between human knowledge and machine inference. If LLMs are not just passive tools but are involved in producing new insights through their inferential processes, are we witnessing the emergence of a new kind of distributed cognition—one that challenges the very foundation of epistemology as a purely human domain?
Where Do We Go From Here?
The nature of epistemology in the era of LLMs challenges us to rethink what it means to know. If LLMs can infer patterns and create outputs that mimic human knowledge, does that diminish the role of human understanding? Or does it expand the boundaries of how we define knowledge in a world where machines increasingly participate in knowledge production?
Perhaps we’re entering an era of complementary epistemology, where human understanding and machine inference coexist, each contributing to a broader, more dynamic conception of knowing. Humans bring depth, meaning, and context; machines bring speed, scale, and pattern recognition. Together, they may form a new synthesis of knowledge—one that transcends traditional boundaries and redefines the nature of intelligence itself.
So, what do LLMs really know?
In the end, LLMs don’t "know" anything in the human sense. But their remarkable capacity for inference challenges us to rethink the nature of knowledge itself. As we integrate machine inference more deeply into our systems of understanding, we’re forced to ask: Is knowing really about reflection, belief, and justification—or is it simply about the ability to generate useful, accurate patterns?
As LLMs continue to evolve, the boundary between human knowledge and machine inference may become even more blurred, inviting us to explore new ways of thinking about how we know—and what it means to truly understand.