Artificial Intelligence
AI Identifies Gene Signatures of Cancer Tumors
AI machine learning distinguishes healthy cells from cancer.
Posted June 11, 2022 Reviewed by Vanessa Lancaster
Key points
- Researchers investigated markers of cellular states using a new AI machine learning algorithm.
- The new AI algorithm performed much better than other standard machine learning methods, with an average balanced accuracy of 98 percent.
- Researchers AI machine learning demonstrated the ability to classify epithelial tumors and neuroblastoma accurately.
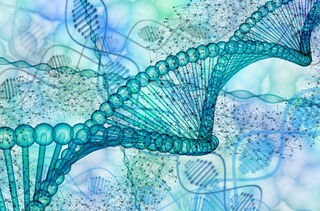
Artificial intelligence (AI) machine learning is emerging as an important tool for medical clinicians and biomedical researchers. A new study published in Genome Biology demonstrates how an AI algorithm has discovered a genetic signature trait of cancer cells, enabling machine learning to distinguish cancer cells from healthy ones.
The researchers set out to discover markers of cellular states using a new AI machine learning algorithm called “ikarus” to compare diverse datasets that have been annotated.
“Here, we propose ikarus, a machine learning pipeline aimed at distinguishing tumor cells from normal cells at the single-cell level,” wrote the researchers affiliated with the Max Delbrück Center for Molecular Medicine in the Helmholtz Association (MDC) and Berlin Institute for Medical Systems Biology. “We test ikarus on multiple single-cell datasets, showing that it achieves high sensitivity and specificity in multiple experimental contexts.”
In biotechnology, omics refers to the study of the structure and functions of a biological function at a specific level, such as the protein level for proteomics, molecular gene level for genomics, and metabolic level for metabolomics.
Research conducted in the various fields of omics often uses groups of cells or tissue samples that contain multiple cells in the quest for biomarkers. This assumes that the cells are the same or uniform in structure or composition or homogeneous. However, in actuality, heterogeneity, or diversity, is inherent in biological organisms. Differences can exist not only in various tissues, organs, and organisms but also within the individual cells in the same tissue or organ itself.
To address this heterogeneity, single-cell sequencing can enable scientists to distinguish genotypes within a group of cells and isolate the cells that have genetic mutations in their genome from the other cells. A single cell is the smallest unit of an organism, from both a functionality and structural standpoint. Single-cell sequencing is the DNA sequencing of just a single cell versus a group of cells in a culture or tissue.
Single-cell sequencing involves isolating the cell, amplifying its genome, then sequencing the DNA. The genome is all of the DNA in an organism, which is the entire set of genetic instructions in a cell that contains all of the information to enable growth and development. DNA sequencing is the process of determining the exact sequence of nucleotide bases in a DNA molecule.
The chemical code that impacts growth and development is determined by the order of the four nucleotide bases that make up DNA. Those four bases are adenine (A), cytosine (C), guanine (G), and thymine (T). The human genome consists of 3.2 billion bases of DNA.
In order to identify genetic markers that are specific to cancer tumors, the team took a two-prong approach. To enable finding gene markers, the researchers consolidated numerous datasets that contain single-cell data that has been already labeled and annotated by experts.
Next, the team trained a logistic regression classifier to discriminate between healthy and cancer cells. Afterward, the team conducted network-based propagation of the cell label (cancer or normal) via a custom-based cell-cell network. The databases used include The Human Protein Atlas, Prognostic Genes, Gene Fusion (ChiTaRs), SEEK (co-expression database), g:Profiler, CancerSEA, MsigDB (GO, Hallmark gene sets), Atlas of co-essential modules, DepMap Achilles scores, and COSMIC.
The new AI algorithm had performed much better than other standard machine learning methods, with an average balanced accuracy of 98 percent. Furthermore, their AI machine learning demonstrated the ability to accurately classify epithelial tumors and neuroblastoma. For comprehensive discrimination of all cancer types, multiple trained models would be needed, according to the researchers. Interestingly, the researchers point out that their AI classifier can be used more broadly for purposes beyond tumor detection to identify any cellular state.
The combination of innovative technologies such as single-cell sequencing in genomics and artificial intelligence machine learning is accelerating the possibility of more targeted, personalized cancer treatment in precision medicine for oncology in the future.
Copyright © 2022 Cami Rosso. All rights reserved.