People’s self-assessments of their own skills, abilities, and test performances are reasonably accurate. Zell & Krizan (2014) report an overall average correlation of 0.3. Whether this value deserves the label ‘reasonably accurate’ depends on a host of assumptions, the context of measurement, and the sampling distribution of the correlation coefficient (see here for more). The correlation coefficient is a statistical index that captures the similarity between two profiles of numbers: the self-assessments of a sample of individuals and their corresponding true scores (or the best scientific measures thereof). Since Galton (1886) introduced the correlation coefficient r (which stands for regression), it has become ubiquitous. Meta-analyses, such as the ones summarized by Zell & Krizan, use it routinely to estimate and express empirical effect sizes (or they use indices like Cohen’s d, which is the difference between two means in standard units, and it is readily translatable into r). In measurement, reliability and validity are also expressed in terms of r.
As a measure of profile similarity, r focuses on one type of similarity between two sets of scores. Self-assessments may also be more variable than true scores, or they may overall be higher (lower). These different types of (dis)similarity can be easily separated from one another (Cronbach & Gleser, 1953; Krueger, 2009). In a commentary on Zell & Krizan, Dunning & Helzer (2014) argue that such separation of scores ought to be done when the accuracy of self-assessment is at stake. They point out that self-assessment contains a constant bias such that estimates are higher on average than are true scores. Other-assessments, i.e., predictions made by peers or observers, are less biased. Correlation coefficients do not capture this difference.
This is a good point, but Dunning and Helzer want more. They urge us to “explore accuracy and error using completely novel measures [and ask] whether subjective prediction or objective performance is most problematic for self-accuracy. Do errors in self-knowledge vary as a function of forecast? Or does the real problem in self-accuracy lie with the objective performance?” (p. 128) They plot (whether these are empirical data, they don’t say) absolute errors (|estimated – actual score|) against predicted performance (which is based on the regression of actual on estimated scores), and then plot absolute errors against actual performance. In the former case, they find a flat horizontal line; in the latter case, they find an asymmetrical U-shaped function with a high branch on the left (low actual scores).
Dunning & Helzer think they have discovered something important. “Self-prediction errors might not be a function of the prediction itself but rather the underlying event that people will subsequently encounter. That is, whether a person is predicting high of low will not tell the researcher whether this person will be more or less likely to be in error” (p. 128-129). Let us first clear up what is probably a misprint. One can predict from people’s predictions whether they are in error. High predictions are more likely to be in error than are low predictions. The mean-level overestimation bias, emphasized by Dunning & Helzer themselves, makes it so. What Dunning and Helzer probably meant to write is that one cannot predict prediction errors from predicted performance, as that is what they graphed. However, the graph simply shows what may be expected from the logic of regression, rather than a discovery. The best-fitting regression line minimizes prediction errors (|actual score – predicted score|). It is mathematically possible that when predicted scores are low, actual scores are more variable than they are when predicted scores are high. This is an unlikely state of affairs, and even if it were observed, its psychological meaning would be opaque.
Dunning & Helzer’s second claim is that actual performance does predict prediction errors. This is not news either. Knowing that the correlation between estimated and actual scores is not perfect (see Zell & Krizan), and knowing that estimates are overall too high, we also know that the prediction errors of the low scorers are greater than the errors of the high scorers (Krueger & Mueller, 2002). Although this pattern of results can be recovered from the logic of regression and the overall tendency for self-overestimation, it has attained some notoriety as a presumably unique phenomenon known as the Dunning-Kruger effect. This treatment of a derivative pattern as a sui-generis phenomenon would not be noteworthy, were it not for its implications. Dunning & Helzer suggest the self-accuracy cannot be improved by helping people make better predictions, but rather by raising their actual scores. The first part of this claim is false. Dunning & Helzer themselves stress the overall, group-level bias of overestimation. It follows that if people were advised to lower their self-assessments by a certain constant, their absolute errors would diminish.
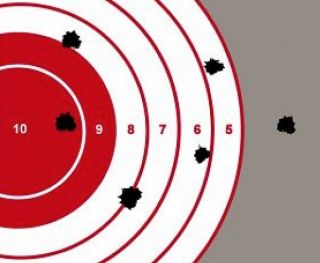
The second part of the claim is also problematic. It is true that if self-predictions remain constant while true scores are raised, absolute errors diminish. This is, however, an instance of the Texas sharpshooter fallacy. Let the predictions be what they are, we bring the criterion – that, which is to be predicted – in line with the prediction, after the fact. The Dunning-Kruger effect suggests that the low performers in particular should be trained to do better. When they do, absolute errors will diminish, but so will the accuracy correlation. As the range of true scores becomes shorter, the remaining random error will be relatively larger than the systematic variability in the true scores. What is gained when one type of accuracy score is improved at the expense of a decrement in another? A situation, in which everyone does well (is rich, healthy, and happy), is socially desirable, but it undermines the value of measurement. Measurement (and science) requires variability.
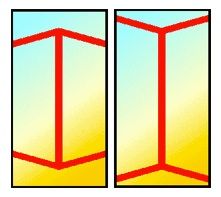
The idea that self-accuracy can be improved by making (low) performers score higher implies a causal claim. Dunning & Helzer think that there is something about low performance that keeps people from seeing the very lowness of their performance. “Poor performers are not in a position to recognize the shortcomings of their performance” (p. 129). Making them perform better “helps them to avoid the type of outcome they seem unable to anticipate” (p. 129). From this causal model, it seems to follow that once people perform better, they will make more accurate predictions. Stated differently, if low performance causes prediction errors, a switch to high performance will eliminate errors. This would be a logically valid modus tollens inference were it not for the fact that low performance is itself part of how error is defined (error = estimated – actual performance). Errors must diminish once performance is raised, even if there is no causal effect whatever.
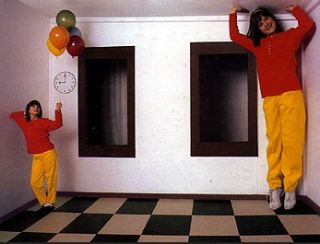
Judgmental errors such as self-enhancement are often treated as if they were cognitive illusions on a par with visual illusions. Most are not, however (Krueger & Funder, 2004), and Dunning & Helzer would have to agree. If they did not agree, they would have to want to correct visual illusions by changing reality. They would have to seek to overcome the Müller-Lyer illusion by making the line with the outbound arrows longer than the line with the inbound arrows; they would have to put a dwarf and a giant in the Ames room; they would have to flip the Necker cube whenever perceptions switch to a different spatial interpretation. The roots of these illusions lie in how the visual system interprets an ambiguous reality. Researchers would have learned less about perception had they never found clever ways of tricking it.
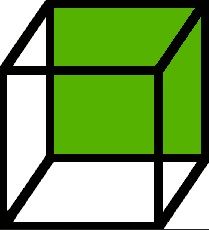
Cronbach, L. J., & Gleser, G. C. (1953). Assessing similarity between profiles. Psychological Bulletin, 50, 456–473.
Dunning, D., & Helzer, E. G. (2014). Beyond the correlation coefficient in studies on self-assessment accuracy. Perspectives on Psychological Science, 92, 126-130.
Galton, F. (1886). Regression towards mediocrity in hereditary stature.The Journal of the Anthropological Institute of Great Britain and Ireland, 15, 246–263.
Krueger, J. I. (2009). A componential model of situation effects, person effects and situation-by-person interaction effects on social behavior. Journal of Research in Personality, 43, 127-136.
Krueger, J. I., & Funder, D. C. (2004). Towards a balanced social psychology: Causes, consequences and cures for the problem-seeking approach to social behavior and cognition. Behavioral and Brain Sciences, 27, 313-327.
Krueger, J., & Mueller, R. A. (2002). Unskilled, unaware, or both? The contribution of social-perceptual skills and statistical regression to self-enhancement biases. Journal of Personality and Social Psychology, 82, 180-188.
Zell, E., & Krizan, Z. (2014). Do people have insight into their abilities? A metasynthesis. Perspectives on Psychological Science, 9, 111-125.