Artificial Intelligence
Researchers Use Advanced AI to Predict Extreme Weather
A new system uses state-of-the-art deep learning techniques for higher accuracy.
Posted February 21, 2020 Reviewed by Davia Sills
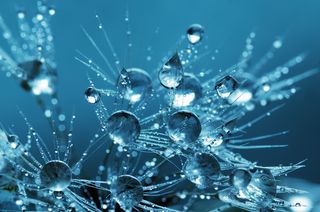
Talk about the weather, generally considered a neutral topic for conversations, is about to get extremely interesting. January 2020 was the Earth’s hottest January in the past 141 years of climate records, according to scientists at NOAA’s National Centers for Environmental Information. Globally, extreme weather and climate disasters pose a threat to public health, economic well-being, and geopolitical stability.
Economically, the U.S. has incurred $1.75 trillion in losses since 1980 due to 258 weather and climate disasters, according to NOAA figures. Predicting extreme weather is a complex science and an area where artificial intelligence (AI) machine learning, specifically the pattern-recognition capabilities of deep learning, can make a difference in forecasting accuracy.
A recent peer-reviewed Rice University study published in February 2020 in the Journal of Advances in Modeling Earth Systems illustrates how an AI system using advanced deep learning techniques can predict extreme weather events up to five days in advance with a high degree of accuracy, using minimal data on current weather conditions.
Capsule neural networks use a set of neurons, or capsule, that are able to process multiple different affine transformations of different objects or object parts simultaneously. In mathematics, affine transformations preserve proportions on lines after transformations, but not necessarily lengths and angles. The output of a capsule is a vector. Cognitive psychologist, computer scientist, and Chief Scientific Advisor of the Vector Institute in Toronto, Geoffrey Hinton, along with Sara Sabour and Nicholas Frosst, introduced the concept of CapsNet in a Neural Information Processing System 2017 conference paper.
Capsule neural networks are capsules added to convolutional neural networks (CNNs). CNNs are deep neural networks frequently used for purposes such as object recognition, natural language processing, and medical image analysis. Unlike traditional neural network layers where every output unit interacts with every input unit, CNNs have sparse interactions and use a specialized linear operation, called a convolution, instead of a general matrix multiplication, in at least one of the layers. Thus, CNNs require storing fewer parameters, and computing the output is more efficient, requiring fewer memory resources.
The Rice researchers focused on extreme hot and cold events, along with their associated onsets, over the North American continent. CapsNet is a deep learning technique that requires supervised training in the form of labeled data. To overcome the complexities of hard-to-label complex weather data, the team created an impact-based auto labeling approach. In this manner, the model could be trained without a complete understanding of the complicated nonlinear relationships between the predictor and the impact of interest.
The researchers reported that their CapsNet system outperformed convolutional neural networks and logistic regression methods. Additionally, the accuracy of the predictions was least impacted when the amount of training set data was decreased.
The Rice researchers have demonstrated a data-driven extreme event prediction framework, thereby laying the foundation for more generalized systems to predict other high-impact climate and environmental events, such as severe air pollution and extreme precipitation in the future.
Copyright © 2020 Cami Rosso All rights reserved.