Cognition
Why I'm All About That Bayes (No Trouble)
Using new statistics in science and everyday life.
Posted June 30, 2023 Reviewed by Abigail Fagan
Key points
- In science and every day life, we may want to make inferences about conclusions or hypotheses.
- People often fail to make good inferences because they don't use Bayes rule, but simple tools can help.
- Decision-makers should adopt Bayesian reasoning for both scientific inference and everyday life.
Whether in everyday life or in the lab, we often want to make inferences about hypotheses. Whether I’m deciding it’s safe to run a yellow light, when I need to leave home in order to make it to my destination on time, or whether the data I collect in the lab supports a particular theory — making decisions in each case requires us to leverage the available information to make good inferences. In doing so, we would like to assess the likelihood of different outcomes and possibilities: will I make the light, will I arrive on time, and is my theory a valid one? Despite their apparent simplicity and the ease with which we can do so intuitively, assigning these likelihoods in a formal, statistical way isn’t entirely straightforward.
One peculiarity spanning most of science is that we often make inferences about data (things that we see in the world) rather than inferences about hypotheses (things we want to predict or assess). The most common approach to statistics in psychology involves calculating how likely it is that we would obtain a particular set of data, given a “null” hypothesis. This null hypothesis is usually an assumption that there is no effect or no relationship between the things we are trying to measure — such as no effect of an experimental drug, or no relationship between income and happiness. If these null hypotheses are true, then the difference between a treatment group and a control group should be close to zero, or the correlation between income and happiness would be close to zero, respectively. If the data seem unlikely (far from zero) under this assumption, then we “reject” the null hypothesis.
The problem with this approach is that there is no way to “accept” or support the null hypothesis. How can we ever decide that a treatment is ineffective, or that there is no relationship between two variables like income and happiness? The traditional approaches we take to statistics mean that we simply cannot make these conclusions. Making the problem worse is the fact that we’re not really making inferences about the interesting hypotheses we actually want to examine — such as how big the effect of the treatment is, or how strong the relationship is — but about a simplistic null hypothesis (is it nonzero?).
If we want to make inferences about hypotheses, we need to turn our analyses around. Null hypothesis testing assigns credibility to data based on simple null assumptions; but more often, we want to assign credibility to hypotheses or possible conclusions based on our data. That’s the whole point of science, after all — to change our understanding of the world (theories, hypotheses) based on the things we observe! So how do we do that?
The answer is given by a formula known as Bayes rule:
Pr(Hypothesis | Data) = Pr(Data | Hypothesis) * Pr(Hypothesis) / Pr(Data)
Don't worry, you don't have to follow all the math. Put simply, we want to assign a probability (Pr) to each hypothesis based on the data we have. This is based on three parts: the likelihood of the data assuming the hypothesis is true (that’s what we covered above!), the likelihood of the data (how often we get the results we got), and our prior beliefs in the hypothesis. These prior beliefs are important: I’m not going to start believing in magic because a magician guesses the card I drew from the magician’s deck, no matter how accurate, because I don’t believe in magic in the first place.
Ironically, people are not always good at making their inferences using Bayes rule. One of the most famous examples comes from assessing the likelihood of breast cancer from mammogram results. The likelihood of having breast cancer given a positive test result is often quite low — if only 1% of people undergoing a mammogram actually have breast cancer, then a positive result from a test that is 90% accurate (comes back positive 90% of the time when breast cancer is present, and negative 90% of the time when it is not present) only results in a true likelihood of breast cancer of about ~8.3%. Yet even physicians will estimate this likelihood at 80-90%! This is referred to as base rate neglect, but it is fundamentally an error where we fail to incorporate prior information: that 1% number (the prior probability of having breast cancer) is really important, because it means that false positives will be much more common than true positives.
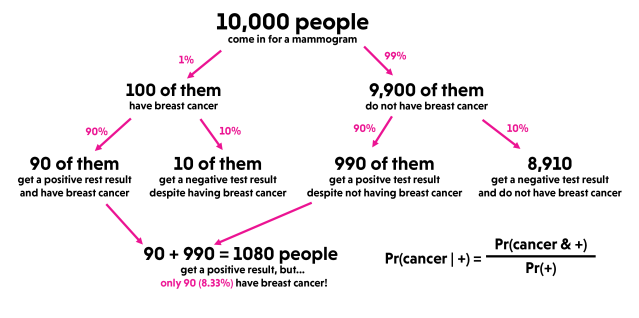
Even when the prior probabilities aren’t an issue, we struggle to reason with Bayes rule. In some of my own work, we showed that people are overly sensitive to the extremeness of the information in front of them (e.g., how strong an opinion piece is written) and relatively insensitive to the reliability of that information (e.g., the credibility of the source, or number of people who agree).
As scientists, we should certainly be using Bayes’ rule to make the best inferences we can — and to make inferences about conclusions or hypotheses (presence of breast cancer, effectiveness of a treatment) rather than merely inferences about data (likelihood of a test result or a pattern of behavior). As everyday people, we should try to use Bayes’ rule when we can. Fortunately, you don’t have be doing math in your head all day — drawing out decision trees like the one I’ve shown above can help you incorporate information like base rates into your inferences.
So if you want to make good inferences as a scientist, consider adopting Bayesian statistics. If you want to make good inferences as a person out there in the world, consider adopting Bayes’ rule and the simple ways to use it (like decision trees)!
References
Barbey, A. K., & Sloman, S. A. (2007). Base-rate respect: From ecological rationality to dual processes. Behavioral and Brain Sciences, 30(3), 241-254.
Kvam, P. D., & Pleskac, T. J. (2016). Strength and weight: The determinants of choice and confidence. Cognition, 152, 170-180.
Gigerenzer, G., & Hoffrage, U. (1995). How to improve Bayesian reasoning without instruction: Frequency formats. Psychological Review, 102(4), 684-704.
Eddy, D. (1982). M.(1982). Probabilistic reasoning in clinical medicine: Problems and opportunities. Judgment under uncertainty: Heuristics and biases, 249-267.